Deep neural network training method based on multiple pre-training
A deep neural network and neural network technology, applied in the field of deep neural network training based on multiple pre-training, can solve problems such as single
- Summary
- Abstract
- Description
- Claims
- Application Information
AI Technical Summary
Problems solved by technology
Method used
Image
Examples
Embodiment
[0108] The following uses a three-hidden-layer neural network as an example to introduce the application of the present invention in a handwritten character recognition system. The network structure is as follows: Figure 4 Shown.
[0109] Step A: Divide the data into training set (containing 50,000 handwritten character pictures), verification set (containing 10,000 handwritten character pictures), and test set (containing 10,000 handwritten character pictures);
[0110] Step B: The size of the unified picture is 24 pixels * 24 pixels;
[0111] Step 2 A: Randomly select the weight matrix W between the input layer and the first hidden layer (1) , Input variable offset b (1) And hidden variable bias c (1) ;
[0112] Second step B: Select 10 samples from the training set as input variables, use the contrast divergence algorithm, and adopt the parameters agreed in the present invention to determine the weight matrix, input variable bias, and implicit value between the input layer and the...
PUM
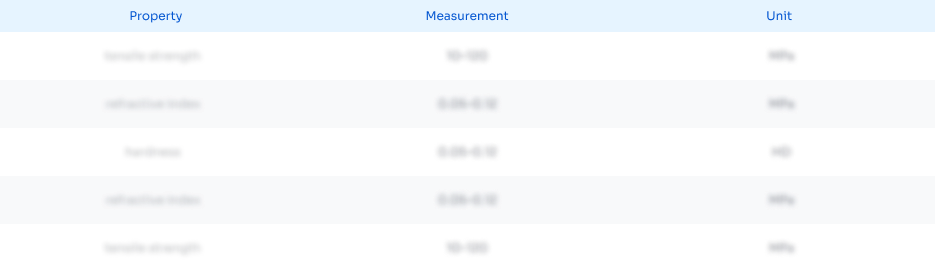
Abstract
Description
Claims
Application Information
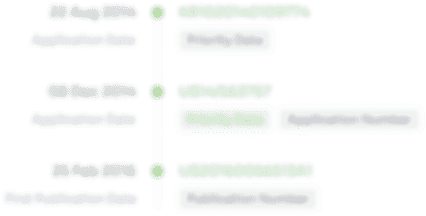
- R&D Engineer
- R&D Manager
- IP Professional
- Industry Leading Data Capabilities
- Powerful AI technology
- Patent DNA Extraction
Browse by: Latest US Patents, China's latest patents, Technical Efficacy Thesaurus, Application Domain, Technology Topic, Popular Technical Reports.
© 2024 PatSnap. All rights reserved.Legal|Privacy policy|Modern Slavery Act Transparency Statement|Sitemap|About US| Contact US: help@patsnap.com