High-spectrum abnormal object detection method based on background dictionary learning and structure sparse expression
A technology of sparse representation and dictionary learning, which is applied in the field of hyperspectral abnormal target detection, can solve the problems of low target detection efficiency and achieve the effect of improving detection rate and accuracy
- Summary
- Abstract
- Description
- Claims
- Application Information
AI Technical Summary
Problems solved by technology
Method used
Image
Examples
Embodiment Construction
[0076] The specific steps of the hyperspectral abnormal target detection method based on background dictionary learning and structural sparse representation of the present invention are as follows:
[0077] Suppose the input hyperspectral image is a 3D data cube containing n b bands, each band is a picture of n row row and n col The column size of the image. For the convenience of calculation, each band is stretched into a row vector, and all row vectors form a two-dimensional matrix X, Among them, each column of X represents the spectrum corresponding to each pixel, and this direction is the spectral dimension; each row of X corresponds to all pixel values of a band (ie n p =n row ×n col ), which is the spatial dimension. The present invention mainly comprises following four steps, specifically as follows:
[0078] 1. Robust background dictionary learning based on principal component analysis.
[0079] (3) Use the double-window local RX algorithm to obtain the back...
PUM
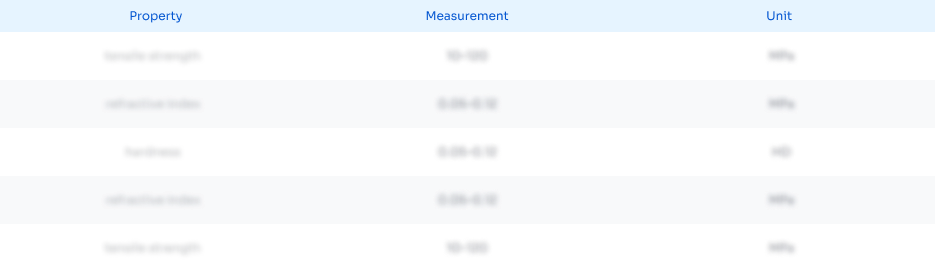
Abstract
Description
Claims
Application Information
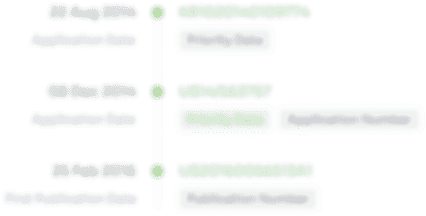
- R&D Engineer
- R&D Manager
- IP Professional
- Industry Leading Data Capabilities
- Powerful AI technology
- Patent DNA Extraction
Browse by: Latest US Patents, China's latest patents, Technical Efficacy Thesaurus, Application Domain, Technology Topic, Popular Technical Reports.
© 2024 PatSnap. All rights reserved.Legal|Privacy policy|Modern Slavery Act Transparency Statement|Sitemap|About US| Contact US: help@patsnap.com