A hyperspectral image unmixing method based on endmember-constrained non-negative matrix factorization
A non-negative matrix decomposition and hyperspectral image technology, which is applied in image analysis, image enhancement, image data processing, etc., can solve the problems that affect the optimal solution acquisition and the existence of local minima, so as to improve the unmixing accuracy and slow down the The effect of mutation
- Summary
- Abstract
- Description
- Claims
- Application Information
AI Technical Summary
Problems solved by technology
Method used
Image
Examples
specific Embodiment approach 1
[0019] The hyperspectral image unmixing method based on endmember constrained non-negative matrix decomposition of this embodiment, combined with figure 1 As shown, the method is realized through the following steps:
[0020] Step 1. Set the pixel spectral vector X, the endmember spectral matrix S, the abundance matrix A of the N-dimensional vector, and the random noise N to establish a linear spectral mixing model:
[0021] X=SA+N (1)
[0022] Wherein, the endmembers refer to various substances contained in the pixels of the hyperspectral image presented by the spectral imager, and these pixels containing the endmembers are called mixed pixels;
[0023] Step 2, using the sum of the absolute values of the pairwise correlation coefficients between the spectra as a correlation function to measure the size of the endmember spectral correlation;
[0024] Step 3, adding the endmember spectrum difference constraint introduced by the natural logarithm function, so that the differ...
specific Embodiment approach 2
[0026] The difference from Embodiment 1 is that in the hyperspectral image unmixing method based on endmember-constrained non-negative matrix decomposition in this embodiment, in the linear spectral mixing model X=SA+N described in step 1, the endmember spectral matrix S= [s 1 ,s 2 ,...,s N ], the element s in the endmember spectral matrix S i Represents the endmember vector, i∈[1,N]; the abundance matrix A of N-dimensional vector=[a 1 ,a 2 ,...,a N ] T , each component element in the abundance matrix A of the N-dimensional vector represents the abundance of the corresponding end member, and
[0027] a i ≥0 (2)
[0028]
[0029] Wherein, the abundance refers to the proportion of one type of end member in the pixel.
specific Embodiment approach 3
[0030] The difference from the specific embodiment 1 or 2 is that in the hyperspectral image unmixing method based on endmember constrained non-negative matrix decomposition in this embodiment, as described in step 2, the sum of the absolute values of the pairwise correlation coefficients between the spectra is used as the correlation The process of measuring the magnitude of the endmember spectral correlation is,
[0031] Step 21. Perform non-negative matrix decomposition based on endmember constraints:
[0032] Using the NMF algorithm, by minimizing the Euclidean distance objective function, the optimal solution of S and A is obtained when X is known.
[0033]
[0034] The iteration formula is:
[0035] S←S-β 1 (SA-X)A T (5)
[0036] A←A-β 2 S T (SA-X) (6)
[0037] The NMF-based spectral unmixing algorithm does not need to determine whether there is a pure pixel, and obtains the abundance of the corresponding endmember while extracting the endmember;
[0038] I...
PUM
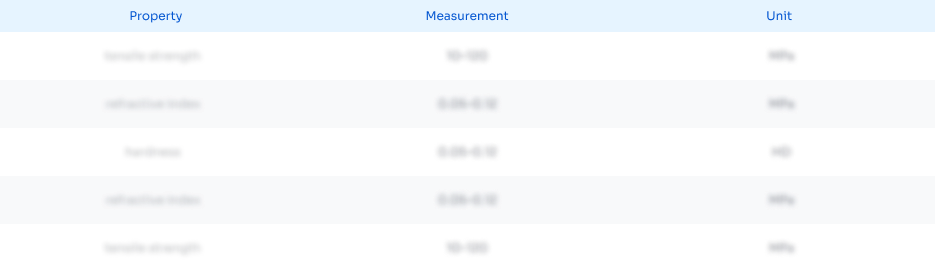
Abstract
Description
Claims
Application Information
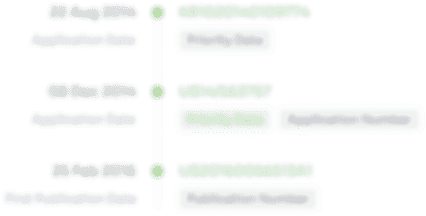
- R&D Engineer
- R&D Manager
- IP Professional
- Industry Leading Data Capabilities
- Powerful AI technology
- Patent DNA Extraction
Browse by: Latest US Patents, China's latest patents, Technical Efficacy Thesaurus, Application Domain, Technology Topic, Popular Technical Reports.
© 2024 PatSnap. All rights reserved.Legal|Privacy policy|Modern Slavery Act Transparency Statement|Sitemap|About US| Contact US: help@patsnap.com