Unbalanced data sampling method in improved C4.5 decision tree algorithm
A data sampling and decision tree technology, applied in the field of data processing, can solve problems such as the difficulty of accurately estimating the cost of misclassification
- Summary
- Abstract
- Description
- Claims
- Application Information
AI Technical Summary
Problems solved by technology
Method used
Image
Examples
Embodiment
[0054] Using the two-month user replacement data set of an operator as the research object, the number of replacement users per month is far less than that of non-replacement users. Effectively predicting replacement users and taking corresponding marketing measures can bring benefits to the company. Very profitable. The learning set is the data records of a telecom operator distributed according to the natural ratio of 200,000 in April (non-replacement: replacement = 27:1), and the test set is the data records of 400,000 in May according to the distribution of 1:1. Through the combination of feature selection and expert experience, 19 attributes are selected as the input features of the prediction model. In addition, in view of the fact that the attributes are independent of each other in the learning process, but in the actual situation, the user’s contribution income, call Time and traffic are closely related, so 9 attributes are artificially added to measure the changes of...
PUM
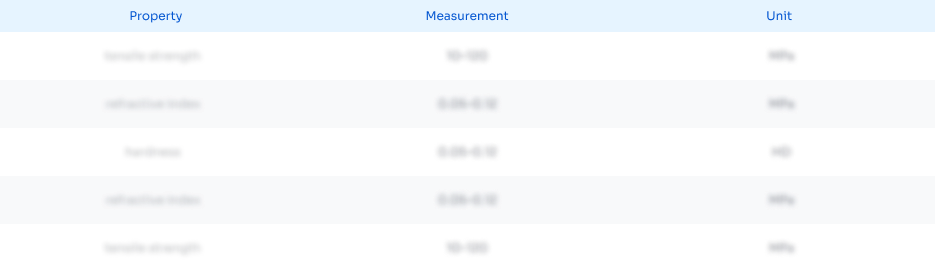
Abstract
Description
Claims
Application Information
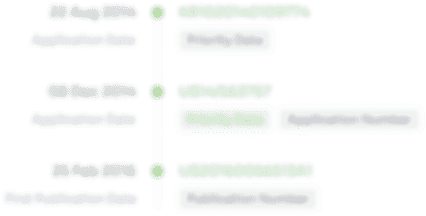
- R&D Engineer
- R&D Manager
- IP Professional
- Industry Leading Data Capabilities
- Powerful AI technology
- Patent DNA Extraction
Browse by: Latest US Patents, China's latest patents, Technical Efficacy Thesaurus, Application Domain, Technology Topic, Popular Technical Reports.
© 2024 PatSnap. All rights reserved.Legal|Privacy policy|Modern Slavery Act Transparency Statement|Sitemap|About US| Contact US: help@patsnap.com