Large-compression-ratio satellite remote sensing image compression method based on deep self-encoding network
A self-encoding network and satellite remote sensing technology, which is applied in the field of satellite remote sensing image compression and remote sensing image compression with a large compression ratio, can solve the problem of not being able to dig out the hidden explanatory factors of complex unstructured scenes, and reduce the cost of application processing Time, easy to achieve, good real-time effect
- Summary
- Abstract
- Description
- Claims
- Application Information
AI Technical Summary
Problems solved by technology
Method used
Image
Examples
Embodiment 1
[0033] The present invention is a large compression ratio satellite remote sensing image compression method based on deep self-encoding network, see figure 1 , including the following steps:
[0034] 1) Construct a deep autoencoder network, and stack multiple autoencoders in cascade to form a deep autoencoder network. The autoencoder mainly includes basic autoencoders, sparse autoencoders, noise reduction autoencoders, and regularized autoencoders. . The invention utilizes the technical advantages of the deep learning technology to gradually extract high-order sparse features of data, constructs a deep self-encoding network to extract high-order sparse features of remote sensing images, and applies it to satellite remote sensing image compression.
[0035] 2) Train the deep self-encoding network, input a set of training image data to the deep self-encoding network, train the network to obtain optimized network parameters, and obtain the deep compression network and deep decom...
Embodiment 2
[0040] The large compression ratio satellite remote sensing image compression method based on deep self-encoding network is the same as the multiple self-encoders described in step 1) in embodiment 1, and the number should be selected within the range of 2-9. Theoretically, the number of autoencoders is unlimited, but too many autoencoders make the network structure more complicated, and the number of samples and time required for training a deep autoencoder network are greatly increased. For multiplier compression, after repeated experiments and comparisons in the present invention, the number of autoencoders preferably ranges from 2 to 9.
[0041]Because the deep autoencoder network is a neural network composed of multiple autoencoders, the output of the previous layer of autoencoder is used as the input of the next layer of autoencoder, so the number of input layer nodes of each autoencoder is related to the hidden The number of layer nodes satisfies: the number of nodes in...
Embodiment 3
[0044] The large compression ratio satellite remote sensing image compression method based on the deep self-encoder network is the same as embodiment 1-2, and the deep compression network in step 3) is: the input layer and the hidden layer of each self-encoder trained are kept connected The relationship and network parameters remain unchanged, and the deep neural network is formed by sequential stacking. That is, the first layer and the second layer of the deep compression network are the input layer and hidden layer of the first autoencoder, the second layer and the third layer are the input layer and hidden layer of the second autoencoder, and the third layer And the fourth layer is the input layer and hidden layer of the third autoencoder, and so on. The number of autoencoders in this example is 2.
[0045] The large compression ratio satellite remote sensing image compression method based on deep self-encoder network is the same as embodiment 1-2, and the deep decompressi...
PUM
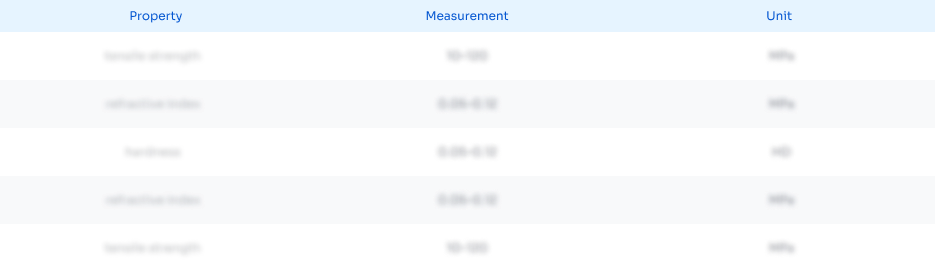
Abstract
Description
Claims
Application Information
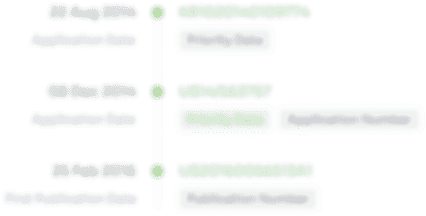
- R&D Engineer
- R&D Manager
- IP Professional
- Industry Leading Data Capabilities
- Powerful AI technology
- Patent DNA Extraction
Browse by: Latest US Patents, China's latest patents, Technical Efficacy Thesaurus, Application Domain, Technology Topic, Popular Technical Reports.
© 2024 PatSnap. All rights reserved.Legal|Privacy policy|Modern Slavery Act Transparency Statement|Sitemap|About US| Contact US: help@patsnap.com