Newly established crossing traffic flow prediction method based on generating type deep belief network
A deep-confidence network and traffic flow technology, applied in the field of traffic flow prediction at new intersections, can solve the problems of less data and low prediction accuracy, and achieve the effect of improving model accuracy and achieving reliability.
- Summary
- Abstract
- Description
- Claims
- Application Information
AI Technical Summary
Problems solved by technology
Method used
Image
Examples
specific Embodiment approach 1
[0026] Specific implementation mode one: the following combination Figure 1 to Figure 3 Illustrate this embodiment, the new intersection traffic flow prediction method based on generation type deep belief network described in this embodiment, it comprises the following steps:
[0027] Step 1: Based on deep learning theory and restricted Boltzmann machine, build a generative deep belief network regression model with 144 input and 144 output structures;
[0028] Step 2: using the mature intersection data of the city to which the new intersection belongs to pre-train the deep belief network regression model to obtain the deep belief network regression pre-training model; the mature intersection is an intersection formed by the accumulation of traffic flow data;
[0029] Step 3: Use the pre-stored actual traffic flow data of the new intersection to continue fine-tuning the deep belief network regression pre-training model, and learn the mapping relationship between the sequences ...
specific Embodiment approach 2
[0032] Specific implementation mode two: the following combination Figure 1 to Figure 3 Describe this embodiment, this embodiment will further explain Embodiment 1, the establishment method of the generative deep belief network regression model in step 1 is:
[0033] Construct a deep belief network regression model with 144 input and 144 output structures through the stacking of restricted Boltzmann machines; the deep belief network regression model is an l-layer neural network, with vector x=h 0 represents the original input, with h 1 ,..., h l-1 Indicates the input of the corresponding hidden layer, h l represents the input of the output layer;
[0034] Among them, the l-1th hidden layer uses a sigmoid function and is composed of a restricted Boltzmann machine, and the top activation function uses a pure linear function;
[0035] For the original input x, the joint probability distribution of l-1 hidden layer and output layer p(x,h 1 ,...,h l )for:
[0036] ...
specific Embodiment approach 3
[0079] Specific implementation mode three: the following combination figure 2 and image 3 Describe this implementation mode. This implementation mode will further explain the implementation mode 2. The specific method for obtaining the final deep belief network regression model in step 3 is:
[0080] Step 31: Use the layer-by-layer greedy method to layer the deep belief network regression pre-training model obtained in step 2, from bottom to top, and then use the pre-stored actual traffic flow data of the new intersection to unsupervised the layer that inputs x training;
[0081] Step 32: After the unsupervised training is over, use supervised learning to fine-tune the deep belief network regression pre-training model;
[0082] Step 33: In addition to the hidden layer of the original input x, use the output of the deep belief network regression pre-training model as a supervisory signal, construct a loss function, and use the gradient descent method to return the deep beli...
PUM
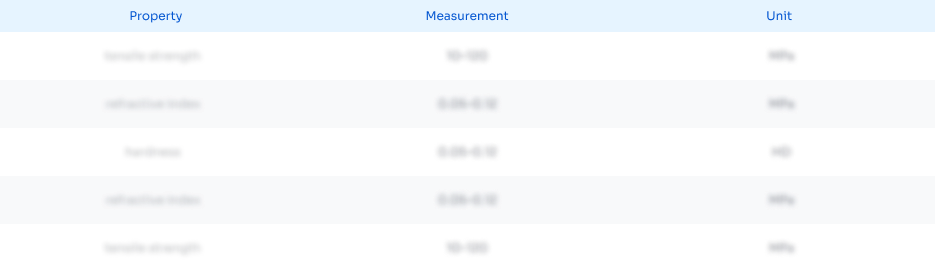
Abstract
Description
Claims
Application Information
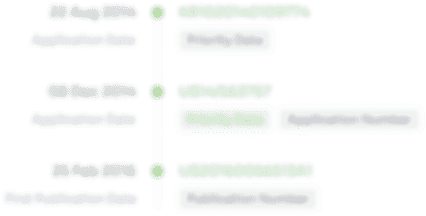
- R&D Engineer
- R&D Manager
- IP Professional
- Industry Leading Data Capabilities
- Powerful AI technology
- Patent DNA Extraction
Browse by: Latest US Patents, China's latest patents, Technical Efficacy Thesaurus, Application Domain, Technology Topic, Popular Technical Reports.
© 2024 PatSnap. All rights reserved.Legal|Privacy policy|Modern Slavery Act Transparency Statement|Sitemap|About US| Contact US: help@patsnap.com