Hyperspectral Image Classification Method Combining Active Learning and Neighborhood Information
A hyperspectral image and active learning technology, applied in the field of hyperspectral image classification combining active learning and neighborhood information, can solve problems such as increased computational workload and redundancy, reduce workload and improve classification accuracy , the effect of improving performance
- Summary
- Abstract
- Description
- Claims
- Application Information
AI Technical Summary
Problems solved by technology
Method used
Image
Examples
Embodiment Construction
[0029] refer to figure 1 , the concrete steps of the present invention are as follows:
[0030] Step 1, use the labeled sample set X l Train the initial SVM classifier.
[0031] Will Figure 4 All the pixels in the hyperspectral image to be classified with a size of m×n are taken as the total sample set X, and 1% of the samples in the total sample set X are randomly selected for expert labeling as the labeled sample set X l , the rest of the sample set X u as an unlabeled sample set, and use the labeled sample set X l Train the initial SVM classifier, set the maximum number of iterations T, T>0, and prepare for the first iteration;
[0032] The SVM method is proposed from the optimal classification surface in the case of linear separability, and it is a method to realize the idea of statistical learning theory. The so-called optimal classification surface requires that the classification surface can not only separate the two categories without errors, but also maximize t...
PUM
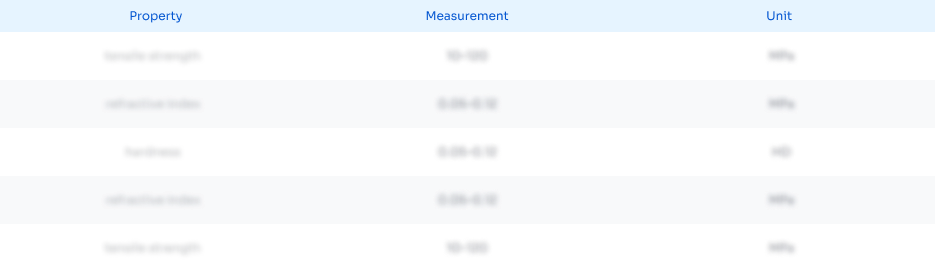
Abstract
Description
Claims
Application Information
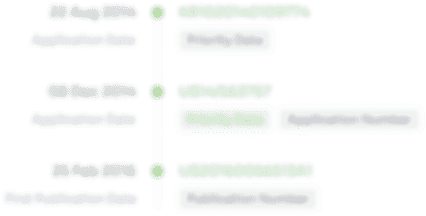
- Generate Ideas
- Intellectual Property
- Life Sciences
- Materials
- Tech Scout
- Unparalleled Data Quality
- Higher Quality Content
- 60% Fewer Hallucinations
Browse by: Latest US Patents, China's latest patents, Technical Efficacy Thesaurus, Application Domain, Technology Topic, Popular Technical Reports.
© 2025 PatSnap. All rights reserved.Legal|Privacy policy|Modern Slavery Act Transparency Statement|Sitemap|About US| Contact US: help@patsnap.com