Unbalance data classifying method based on cluster sampling kernel transformation
A technology of unbalanced data and classification methods, applied in database models, relational databases, electrical digital data processing, etc., can solve problems such as poor classification effects, achieve the effects of reducing data flooding, improving classification effects, and reducing imbalance ratios
- Summary
- Abstract
- Description
- Claims
- Application Information
AI Technical Summary
Problems solved by technology
Method used
Image
Examples
specific Embodiment approach 1
[0028] Specific implementation mode one: combine figure 1 Describe this embodiment, the unbalanced data classification method based on cluster sampling kernel transformation described in this embodiment, it comprises the following steps:
[0029] Step 1: Vectorize the unbalanced data to be classified to obtain an unbalanced data set;
[0030] Step 2: Using the method of cluster sampling based on dynamic self-organizing map to resample the vectors in the unbalanced data set obtained in step 1, and obtain the unbalanced data set after resampling;
[0031] Step 3: Transform the kernel function of the classifier SVM, classify the resampled unbalanced data set obtained in step 2 using the kernel transformed classifier SVM, and obtain the classified unbalanced data set.
[0032] This embodiment mainly solves the classification problem facing the unbalanced data set, and the method realizes the organic combination of the two strategies of sample resampling and classifier improvement...
specific Embodiment approach 2
[0036] Specific embodiment 2: This embodiment is a further limitation of the unbalanced data classification method based on cluster sampling kernel transformation described in specific embodiment 1, using the method of cluster sampling based on dynamic self-organizing map to obtain unbalanced data in step 1 The vectors in the set are resampled, and the methods for obtaining the unbalanced data set after resampling include:
[0037] Step 21: Initialize the self-organizing map network, and set the training times variable cycles to zero;
[0038]Step 22: Initialize the weights of the output layer neuron nodes of the self-organizing map network, and set the weight w of all output layer neuron nodes ij Both assign random decimals, that is, t=0:0ij 1 ,x 2 ,...,x L ) is input to the self-organizing map network, each time a sample is input, the number of training variable cycles is increased by 1, and the total number of input samples is |D|;
[0039] Step 24: Calculate the sample ...
specific Embodiment approach 3
[0059] Specific embodiment three: this embodiment is a further limitation of the unbalanced data classification method based on cluster sampling kernel transformation described in specific embodiment two. In step three, the method for transforming the kernel function of the classifier SVM includes:
[0060] The transformation formula of the kernel function of the classifier SVM is:
[0061] K ~ ( x , x ′ ) = C ( x ) C ( x ′ ) K ( x , x ′ )
[0062] in K ( x , x ′ ...
PUM
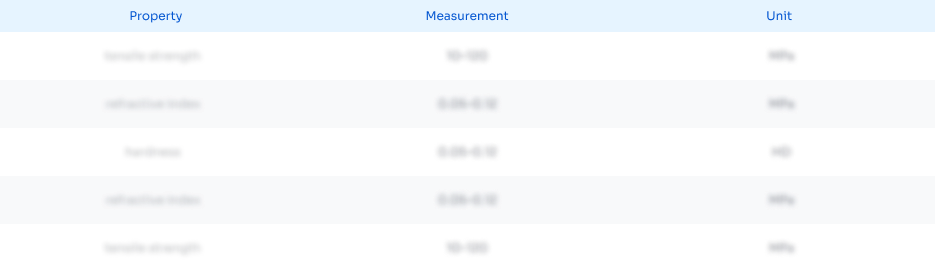
Abstract
Description
Claims
Application Information
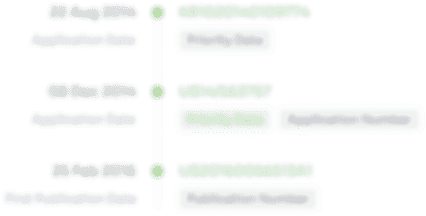
- R&D Engineer
- R&D Manager
- IP Professional
- Industry Leading Data Capabilities
- Powerful AI technology
- Patent DNA Extraction
Browse by: Latest US Patents, China's latest patents, Technical Efficacy Thesaurus, Application Domain, Technology Topic, Popular Technical Reports.
© 2024 PatSnap. All rights reserved.Legal|Privacy policy|Modern Slavery Act Transparency Statement|Sitemap|About US| Contact US: help@patsnap.com