Dynamic hand gesture recognition method based on self incremental learning of hidden Markov model
A technology of dynamic gestures and recognition methods, applied in character and pattern recognition, instruments, computer components, etc., can solve the problems of adjusting model parameters, spending a lot of time and energy, etc., achieve high recognition rate, improve recognition accuracy, and better The effect of robustness
- Summary
- Abstract
- Description
- Claims
- Application Information
AI Technical Summary
Problems solved by technology
Method used
Image
Examples
Embodiment
[0137] In the embodiment, the gesture operation trajectory of 10 Arabic numerals from 0 to 9 is recognized. The operator simulates the trajectories of 10 Arabic numeral strokes in the spatial area with the human hand in front of the camera. These quantized gesture trajectories are used for model training, gesture recognition and incremental learning.
[0138] In the training phase, each Arabic numeral is trained using 40 video streams, that is, 40 training samples, so that the total number of training samples is 400.
[0139] In the recognition stage, the number of recognition samples for each Arabic numeral ranges from 70 to 100 (note: pose "1" was used as a test video during the experiment, so a large number of sample libraries were recorded), and the training samples and recognition The number of samples is shown in Table 1:
[0140] Table 1 List of training samples and recognition samples in the experiment
[0141]
0
1
2
3
4
5
6
...
PUM
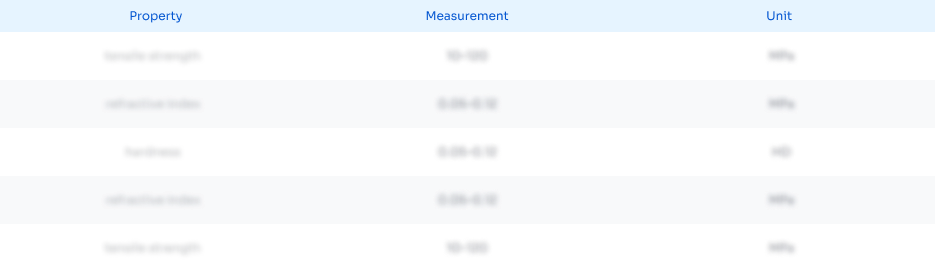
Abstract
Description
Claims
Application Information
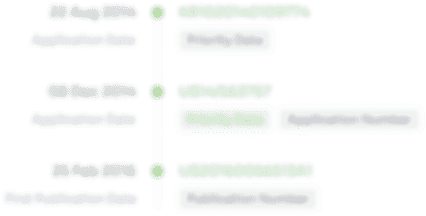
- Generate Ideas
- Intellectual Property
- Life Sciences
- Materials
- Tech Scout
- Unparalleled Data Quality
- Higher Quality Content
- 60% Fewer Hallucinations
Browse by: Latest US Patents, China's latest patents, Technical Efficacy Thesaurus, Application Domain, Technology Topic, Popular Technical Reports.
© 2025 PatSnap. All rights reserved.Legal|Privacy policy|Modern Slavery Act Transparency Statement|Sitemap|About US| Contact US: help@patsnap.com