Spatial Fisher vector based image classification method
A classification method and image technology, applied in the field of image processing, can solve problems such as the inability to describe the location range, the lack of consideration of the spatial layout information of feature points, and the disorder.
- Summary
- Abstract
- Description
- Claims
- Application Information
AI Technical Summary
Problems solved by technology
Method used
Image
Examples
Embodiment Construction
[0030] The solutions and effects of the present invention will be further described in detail below in conjunction with the accompanying drawings.
[0031] refer to figure 1 , the implementation steps of the present invention are as follows:
[0032] Step 1, divide the image set M to be classified into a training set M 1 and the test set M 2 , to extract the "scale-invariant feature transformation" feature points of all images in the image set M.
[0033] The implementation of this step can use the existing scale-invariant feature conversion method, SURF method and Daisy method. In this example, the scale-invariant feature conversion method is used. The steps are as follows:
[0034] 1a) Use the Gaussian convolution kernel to generate the Gaussian difference scale space D(x,y,σ) of an image in the image set M:
[0035] D(x,y,σ)=(G(x,y,kσ)-G(x,y,σ))*I(x,y),
[0036] Among them, * represents the convolution operation, I(x,y) represents the image in the image set M, σ repres...
PUM
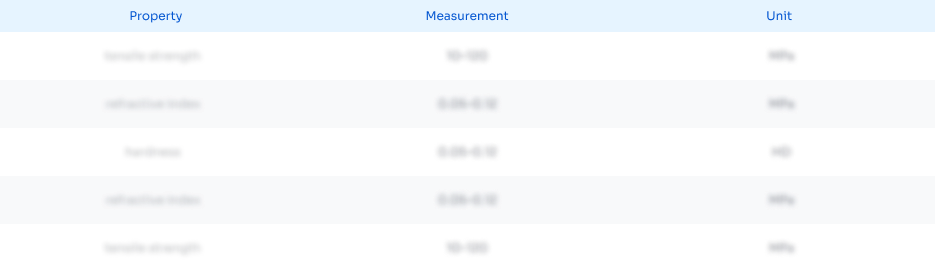
Abstract
Description
Claims
Application Information
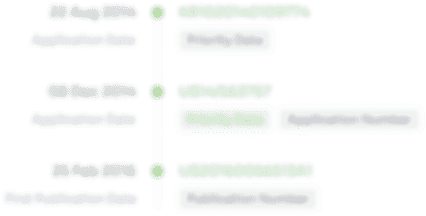
- R&D Engineer
- R&D Manager
- IP Professional
- Industry Leading Data Capabilities
- Powerful AI technology
- Patent DNA Extraction
Browse by: Latest US Patents, China's latest patents, Technical Efficacy Thesaurus, Application Domain, Technology Topic, Popular Technical Reports.
© 2024 PatSnap. All rights reserved.Legal|Privacy policy|Modern Slavery Act Transparency Statement|Sitemap|About US| Contact US: help@patsnap.com