Method for extracting classification rule based on fuzzy-rough model
A fuzzy and rough, extraction method technology, applied in special data processing applications, instruments, electrical digital data processing and other directions, can solve the problems of continuous attribute value fuzzy boundary not taking into account, complex data mining process, loss of important data information, etc.
- Summary
- Abstract
- Description
- Claims
- Application Information
AI Technical Summary
Problems solved by technology
Method used
Image
Examples
Embodiment
[0044] A method for extracting classification rules based on a fuzzy rough model, comprising:
[0045] 1. Fuzzification of continuous attributes
[0046] (1) Decision-making system for continuous attribute values
[0047] Suppose a decision system (U, Q, V, f), where U={x 1 , x 2 ,Λ,x n} is a non-empty finite universe, representing an object; Q is a non-empty attribute set, Q=CY{d}, C={q 1 ,q 2 ,Λ,q m} is a non-empty, finite conditional attribute set, {d} is a decision attribute set, d: U→{1, 2, Λ, g}; V is an attribute value, V=V c YV d , V C ={V q : q∈C} is the set of conditional attribute values, V d is the decision attribute value set, and the attribute value v of the i-th object under the j-th conditional attribute ij (1=1Λn, j=1Λm) is a continuous attribute value; f: U×Q→V is an information mapping function, obviously this is a decision system with continuous attribute value.
[0048] (2) Attribute fuzzification
[0049] In practical applications, the key to...
PUM
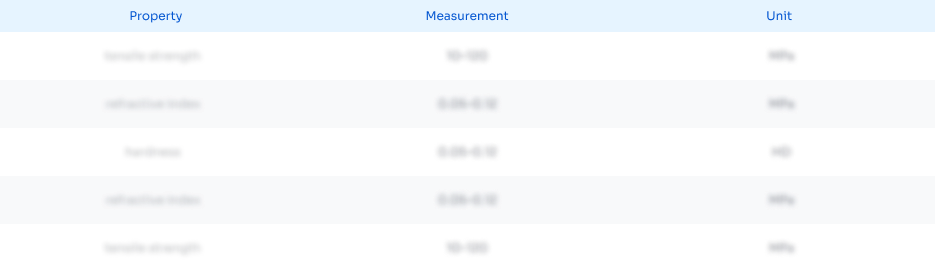
Abstract
Description
Claims
Application Information
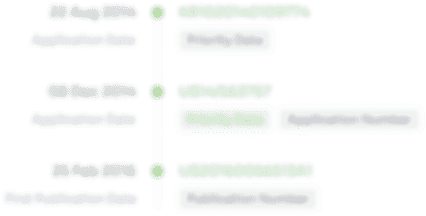
- R&D Engineer
- R&D Manager
- IP Professional
- Industry Leading Data Capabilities
- Powerful AI technology
- Patent DNA Extraction
Browse by: Latest US Patents, China's latest patents, Technical Efficacy Thesaurus, Application Domain, Technology Topic, Popular Technical Reports.
© 2024 PatSnap. All rights reserved.Legal|Privacy policy|Modern Slavery Act Transparency Statement|Sitemap|About US| Contact US: help@patsnap.com