Battery charge state estimation method based on minimum error entropy extended Kalman filtering
A battery state of charge, extended Kalman technology, applied in the measurement of electricity, electrical components, measurement of electrical variables and other directions, can solve the problems of low accuracy, singular values, not suitable for online estimation, etc., to achieve strong robustness, High precision, ensure safe use and efficient management
- Summary
- Abstract
- Description
- Claims
- Application Information
AI Technical Summary
Problems solved by technology
Method used
Image
Examples
Embodiment Construction
[0086] The present invention will be further described below with reference to the accompanying drawings and embodiments.
[0087] Taking the initial value of SOC as 1 and the measurement noise in a complex non-Gaussian environment as an example, the battery state of charge estimation method based on the minimum error entropy extended Kalman filter includes the following steps:
[0088] Step 1: Build the battery equivalent circuit like figure 1 shown. The model consists of the following parts: (1) an ideal voltage source, representing the open-circuit voltage U oc ; (2) R0 represents the ohmic internal resistance of the battery (3) R e C e Used to model the electrochemical polarization of the battery, R d C d Used to represent the concentration polarization inside the cell. i is the charging and discharging current of the battery, U b is the battery terminal voltage, and the time constant of the two RC loops is τ e =R e C e , τ d =R d C d .
[0089] Step 2: Sele...
PUM
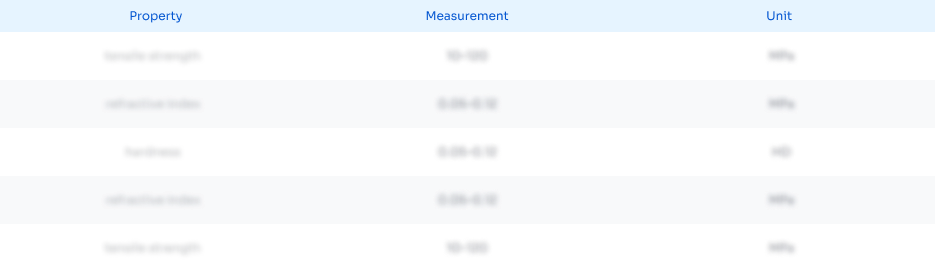
Abstract
Description
Claims
Application Information
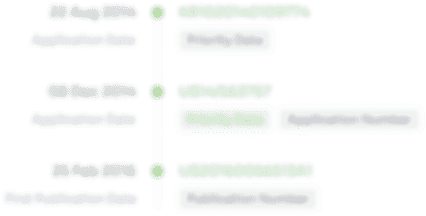
- R&D Engineer
- R&D Manager
- IP Professional
- Industry Leading Data Capabilities
- Powerful AI technology
- Patent DNA Extraction
Browse by: Latest US Patents, China's latest patents, Technical Efficacy Thesaurus, Application Domain, Technology Topic, Popular Technical Reports.
© 2024 PatSnap. All rights reserved.Legal|Privacy policy|Modern Slavery Act Transparency Statement|Sitemap|About US| Contact US: help@patsnap.com