Severe pancreatitis prediction model construction method based on machine learning method
A prediction model and machine learning technology, applied in the field of disease diagnosis, can solve the problems of high workload of doctors, subjective bias, general repeatability, etc., and achieve the effect of fast prediction speed, resolution of diagnosis lag, and high accuracy
- Summary
- Abstract
- Description
- Claims
- Application Information
AI Technical Summary
Problems solved by technology
Method used
Image
Examples
Embodiment
[0050] Such as Figure 1-Figure 2 As shown, a method for constructing a severe pancreatitis prediction model based on machine learning methods, the construction steps are as follows:
[0051] S1. Obtain the discharge diagnosis result and clinical relevant data of the predicted object, and preprocess the obtained data;
[0052] S2. Screen the preprocessed data and bin the data, and combine the binned data with the unbinned data;
[0053] S3. Establish multiple severe pancreatitis prediction models based on binned data and unbinned data and compare them to select an excellent model, and construct a predictive receiver operating characteristic curve;
[0054] S4. Pre-train the selected model and screen the 10 feature indicators with the largest contribution to the model, and use the 10 feature indicators with the largest contribution to the model screened by the pre-trained model to perform retraining;
[0055] S5. Confirm the threshold value range for evaluating severe pancrea...
PUM
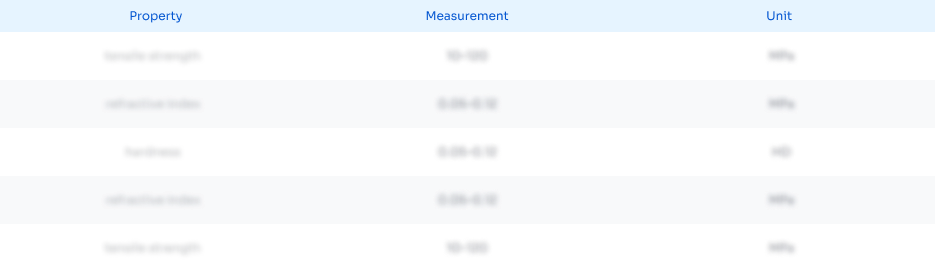
Abstract
Description
Claims
Application Information
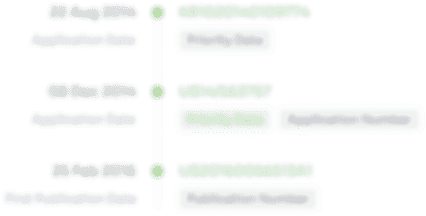
- R&D Engineer
- R&D Manager
- IP Professional
- Industry Leading Data Capabilities
- Powerful AI technology
- Patent DNA Extraction
Browse by: Latest US Patents, China's latest patents, Technical Efficacy Thesaurus, Application Domain, Technology Topic.
© 2024 PatSnap. All rights reserved.Legal|Privacy policy|Modern Slavery Act Transparency Statement|Sitemap