Distributed traffic signal control method based on generative adversarial network and reinforcement learning
A reinforcement learning and traffic signal technology, which is applied in the traffic control system of road vehicles, traffic signal control, traffic control system, etc., can solve the problems of independent operation, low data accumulation efficiency, and poor regional joint control effect, etc., to achieve Improve learning ability and solve the effect of low generation efficiency
- Summary
- Abstract
- Description
- Claims
- Application Information
AI Technical Summary
Problems solved by technology
Method used
Image
Examples
Embodiment Construction
[0055] The present invention will be further described below in conjunction with the accompanying drawings and specific embodiments.
[0056] A distributed traffic signal control method based on generative confrontation network and reinforcement learning disclosed in the embodiment of the present invention is suitable for distributed signal control of regional road traffic. It mainly includes modeling the road traffic environment to define the three elements of agent reinforcement learning (state, action and reward); the agent interacts with the simulation environment to accumulate experience database A policy-based generative adversarial model (P-WGAN-GP) is then constructed and trained to generate a pseudo-database Finally, the reinforcement learning A2C model is constructed, and the interaction mode between generative confrontation model and reinforcement learning is proposed, and the experience database and pseudo-database are used for model parameter training. Specific...
PUM
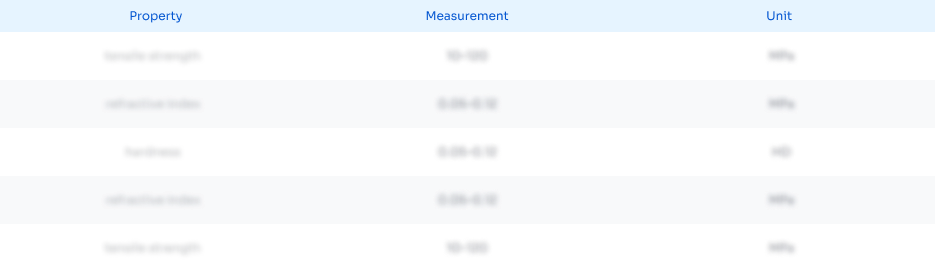
Abstract
Description
Claims
Application Information
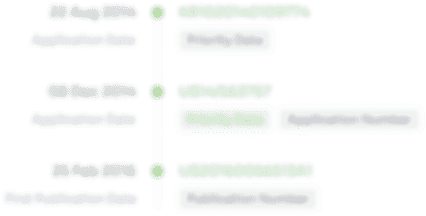
- Generate Ideas
- Intellectual Property
- Life Sciences
- Materials
- Tech Scout
- Unparalleled Data Quality
- Higher Quality Content
- 60% Fewer Hallucinations
Browse by: Latest US Patents, China's latest patents, Technical Efficacy Thesaurus, Application Domain, Technology Topic, Popular Technical Reports.
© 2025 PatSnap. All rights reserved.Legal|Privacy policy|Modern Slavery Act Transparency Statement|Sitemap|About US| Contact US: help@patsnap.com