Sample extraction and expansion method for small sample image recognition, and storage medium
A technology of image recognition and sample expansion, applied in the field of image processing, can solve the problems of generating wrong samples and affecting the training effect, and achieve the effect of stable effect, improved recognition effect and accurate data
- Summary
- Abstract
- Description
- Claims
- Application Information
AI Technical Summary
Problems solved by technology
Method used
Image
Examples
specific Embodiment approach 1
[0050] This embodiment is a sample extraction method for small-sample image recognition, which is essentially a sampling method based on feature reconstruction. Its main idea is to optimally divide large-sample data through unsupervised fuzzy clustering. Then reconstruct the typical small sample data set. Specifically, the following steps are included:
[0051] First, calculate the central support point C of each category of the image large sample data set k .
[0052] Secondly, from the perspective of sample characteristics, the same kind of sample data is divided into dynamic number of clusters, and the centroid of each cluster is calculated according to each division situation.
[0053] Then, according to the centroid of each cluster, calculate the new center point of the category in this division. And by calculating the sum of the error of the same cluster and the error of the centroid as the total error of the category in this division. The case with the smallest erro...
specific Embodiment approach 2
[0076] This embodiment is a storage medium, and at least one instruction is stored in the storage medium, and the at least one instruction is loaded and executed by a processor to implement a sample extraction method for small-sample image recognition.
specific Embodiment approach 3
[0078] This embodiment is a sample expansion method for small-sample image recognition, which is essentially a sample expansion method based on intra-class deformation information. Its main idea is to generate and expand a new data set of this category by learning the deformation information between the same category of data, and then using the deformation information on other samples of the same category. In order to better learn the deformation information between similar data, through the sample extraction method of feature reconstruction, the specific implementation mode 1 provides a typical small-sample data set with relatively complete features for data expansion, and each category in the typical small-sample data set All are divided into multi-clusters with characteristic differences, and then expanded based on the small sample data set determined in the first embodiment. figure 1 It is an overview diagram of the sample extraction and expansion method based on feature r...
PUM
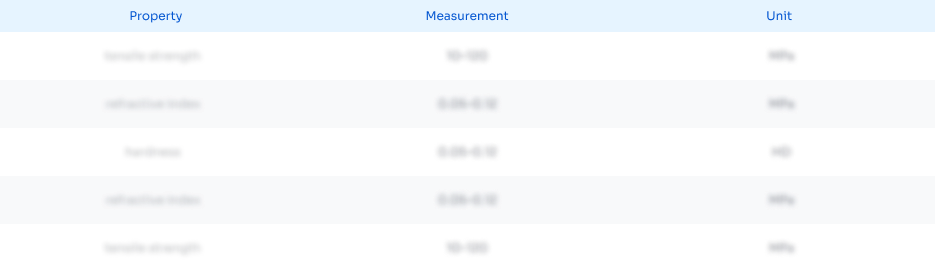
Abstract
Description
Claims
Application Information
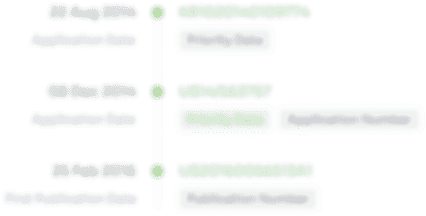
- R&D
- Intellectual Property
- Life Sciences
- Materials
- Tech Scout
- Unparalleled Data Quality
- Higher Quality Content
- 60% Fewer Hallucinations
Browse by: Latest US Patents, China's latest patents, Technical Efficacy Thesaurus, Application Domain, Technology Topic, Popular Technical Reports.
© 2025 PatSnap. All rights reserved.Legal|Privacy policy|Modern Slavery Act Transparency Statement|Sitemap|About US| Contact US: help@patsnap.com