Anomaly Monitoring Method for Uncertain Nonstationary Industrial Processes
An uncertainty and anomaly monitoring technology, applied to instruments, complex mathematical operations, calculations, etc., to improve detection capabilities and avoid over-fitting problems
- Summary
- Abstract
- Description
- Claims
- Application Information
AI Technical Summary
Problems solved by technology
Method used
Image
Examples
Embodiment 1
[0191] What is tested in embodiment 1 is the heat transfer coefficient degeneration fault, Figure 3-Figure 8 The monitoring results of different algorithms are summarized. The fault is a typical small fault. In the early stage of the fault, the three methods based on cointegration analysis, stationary subspace analysis and probabilistic stationary subspace analysis all showed different degrees of underreporting. Statistics have the shortest detection latency, such as Figure 7 shown. The advantage of statistics is that they contain non-stationary information. Additionally, if Figure 4 and Figure 5 As shown, the recursive principal component analysis method gives an earlier alarm in the early stage of the fault, but leaves many false alarms as the magnitude of the fault increases. This reflects the inability of the recursive principal component analysis method to discern minor faults from non-stationary trends.
Embodiment 2
[0193] In Example 2, an additive constant sensor deviation fault, which occurs with an amplitude of 1 in the reaction temperature variable, was tested. Compared with the nominal value of the reaction temperature of 430°C, this is a small fault with a smaller magnitude. In this example, the monitoring results of four different methods are as follows Figure 9-Figure 14 shown. from Figure 9 and Figure 12 It can be seen that the methods based on cointegration analysis and stationary subspace analysis can only partially detect the fault, but leave a certain degree of false positives. Both methods fail to model non-stationary components, thus resulting in unsatisfactory monitoring performance for small faults. In addition, the recursive principal component analysis method can hardly detect the failure (see Figure 10 and Figure 11 ), because the fault magnitude is too small to be masked by the non-stationary trend. For probabilistic stationary subspace analysis, the The...
PUM
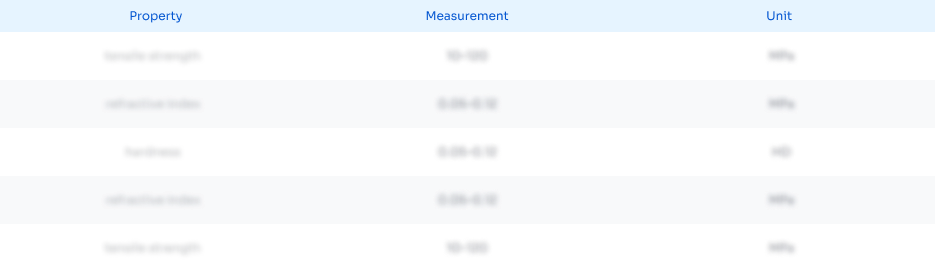
Abstract
Description
Claims
Application Information
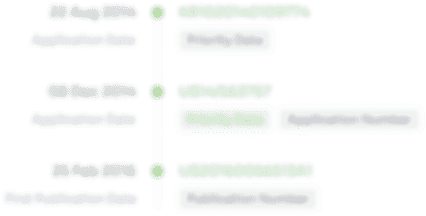
- R&D Engineer
- R&D Manager
- IP Professional
- Industry Leading Data Capabilities
- Powerful AI technology
- Patent DNA Extraction
Browse by: Latest US Patents, China's latest patents, Technical Efficacy Thesaurus, Application Domain, Technology Topic, Popular Technical Reports.
© 2024 PatSnap. All rights reserved.Legal|Privacy policy|Modern Slavery Act Transparency Statement|Sitemap|About US| Contact US: help@patsnap.com