Anti-unmanned aerial vehicle task allocation method based on reinforcement learning
A technology of reinforcement learning and task assignment, applied in neural learning methods, stochastic CAD, design optimization/simulation, etc., to achieve the effects of good robustness, efficient and accurate assignment results, and saving convergence time
- Summary
- Abstract
- Description
- Claims
- Application Information
AI Technical Summary
Problems solved by technology
Method used
Image
Examples
Embodiment Construction
[0051] In order to make the object, technical solution and advantages of the present invention clearer, the present invention will be further described in detail below in conjunction with the accompanying drawings. Obviously, the described embodiments are only some embodiments of the present invention, rather than all embodiments . Based on the embodiments of the present invention, all other embodiments obtained by persons of ordinary skill in the art without making creative efforts belong to the protection scope of the present invention.
[0052] It should be understood that the specific embodiments described here are only used to explain the present invention, not to limit the present invention.
[0053] The present invention uses a simulation scene to test the performance of the algorithm. The simulation scene refers to the size of domestic airports and the building environment. Considering the resource cost of firepower deployment and the size of the airport, 9 intercepti...
PUM
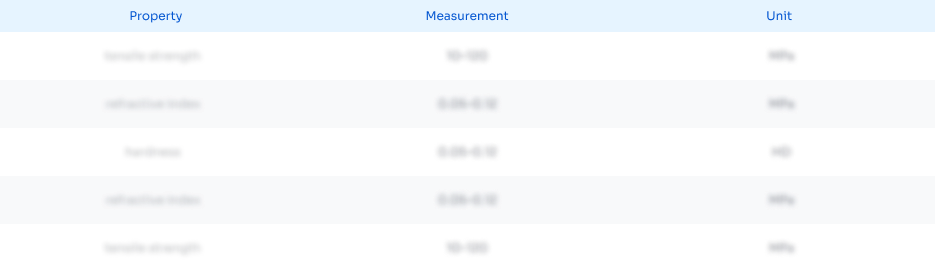
Abstract
Description
Claims
Application Information
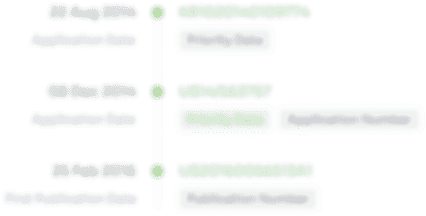
- R&D Engineer
- R&D Manager
- IP Professional
- Industry Leading Data Capabilities
- Powerful AI technology
- Patent DNA Extraction
Browse by: Latest US Patents, China's latest patents, Technical Efficacy Thesaurus, Application Domain, Technology Topic, Popular Technical Reports.
© 2024 PatSnap. All rights reserved.Legal|Privacy policy|Modern Slavery Act Transparency Statement|Sitemap|About US| Contact US: help@patsnap.com