Active learning short text classification method and system based on sampling frequency optimization
A sampling frequency, active learning technology, applied in neural learning methods, text database clustering/classification, unstructured text data retrieval, etc. Direction, effect on a wide range of applications
- Summary
- Abstract
- Description
- Claims
- Application Information
AI Technical Summary
Problems solved by technology
Method used
Image
Examples
Embodiment Construction
[0037] The present invention will be described in detail below in conjunction with the accompanying drawings and specific embodiments. Note that the aspects described below in conjunction with the drawings and specific embodiments are only exemplary, and should not be construed as limiting the protection scope of the present invention.
[0038] Before describing the technical solution of the present invention, the principle of the active learning short text classification of the present invention will be described first.
[0039] For the active learning framework, it is assumed that a given data set z={(x 1 ,y 1 ),...(x N ,y N )}, where x i is a D-dimensional feature vector, y i ∈{0,1,...,K}, the above-mentioned N, K, and D are certain constants. The data set z is divided into labeled data sets L t and the unlabeled dataset U t .
[0040] Active learning short text classification algorithms generally include the following steps:
[0041] a. A small fraction of labele...
PUM
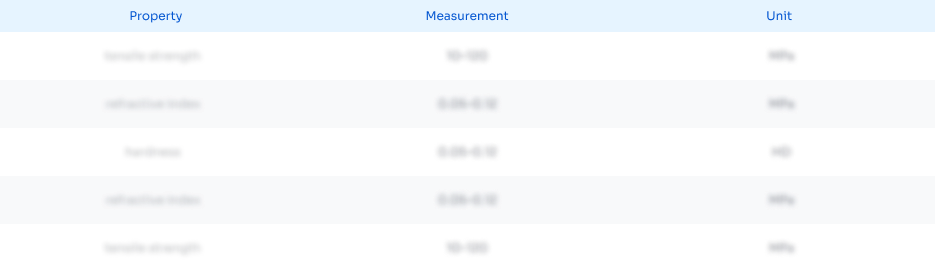
Abstract
Description
Claims
Application Information
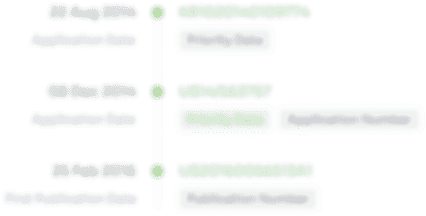
- R&D Engineer
- R&D Manager
- IP Professional
- Industry Leading Data Capabilities
- Powerful AI technology
- Patent DNA Extraction
Browse by: Latest US Patents, China's latest patents, Technical Efficacy Thesaurus, Application Domain, Technology Topic, Popular Technical Reports.
© 2024 PatSnap. All rights reserved.Legal|Privacy policy|Modern Slavery Act Transparency Statement|Sitemap|About US| Contact US: help@patsnap.com