Multi-view low-rank sparse subspace clustering method based on exclusive regularization
A clustering method and exclusive technology, applied in the field of data processing, can solve the problem of low multi-view clustering performance, and achieve the effect of ensuring low rank and sparsity
- Summary
- Abstract
- Description
- Claims
- Application Information
AI Technical Summary
Problems solved by technology
Method used
Image
Examples
Embodiment Construction
[0017] refer to figure 1 , the specific implementation process of the present invention is as follows:
[0018] Step 1. For the given multi-view data X, according to the low-rank sparse subspace clustering method, low-rank and sparse constraints are respectively performed on the representation coefficient matrix Z of each view data. The model is
[0019]
[0020] s.t.X (v) =X (v) Z (v) +E (v) ,diag(Z (v) )=0,
[0021] where Z (v) ∈ R N×N ,E (v) ∈ R D×N Indicates the noise of the data, λ 1 ,λ 2 are non-negative low-rank, sparse regularization parameters, respectively.
[0022] Step 2, for different view data, pairwise exclusive constraints are imposed on the representation coefficient matrix Z respectively. Its exclusive constraints are expressed as where is the Hadamard product. due to l 0 The norm is non-convex, so use its convexity instead to change the above constraint to The purpose of this constraint is to make the data in different views as diverse ...
PUM
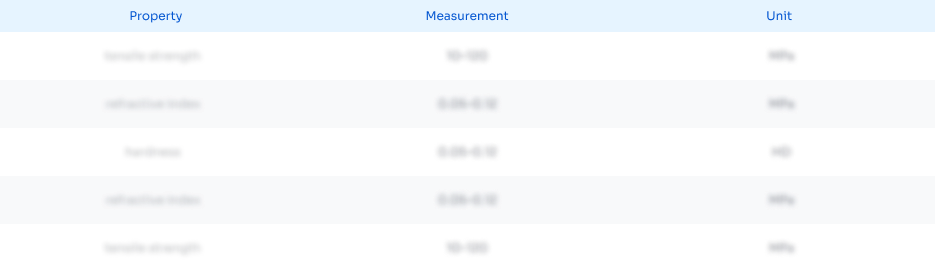
Abstract
Description
Claims
Application Information
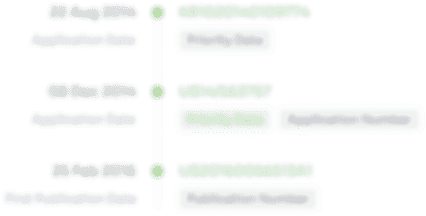
- R&D Engineer
- R&D Manager
- IP Professional
- Industry Leading Data Capabilities
- Powerful AI technology
- Patent DNA Extraction
Browse by: Latest US Patents, China's latest patents, Technical Efficacy Thesaurus, Application Domain, Technology Topic, Popular Technical Reports.
© 2024 PatSnap. All rights reserved.Legal|Privacy policy|Modern Slavery Act Transparency Statement|Sitemap|About US| Contact US: help@patsnap.com