A no-reference video quality assessment method based on feature fusion and recurrent neural network
A technology of cyclic neural network and feature fusion, which is applied in TV, electrical components, image communication, etc., can solve problems such as poor quality evaluation performance, and achieve the effect of large detection quality range, accurate quality evaluation, and accurate quality evaluation indicators.
- Summary
- Abstract
- Description
- Claims
- Application Information
AI Technical Summary
Problems solved by technology
Method used
Image
Examples
Embodiment approach
[0047] The flow chart of the implementation is as figure 1 shown, including the following steps:
[0048] Step S10, extracting and cropping video segments;
[0049] Step S20, building and training a feature fusion network;
[0050] Step S30, obtaining the feature vector representation of the video;
[0051] Step S40, building and training a recurrent neural network;
[0052] Step S50, evaluating the quality of the video;
[0053] The extraction and cropping video segment adjustment step S10 of the embodiment also includes the following steps:
[0054] Step S100, extracting video frames, selecting video frames at equal intervals, and discarding other video frames directly due to redundancy;
[0055] Step S110, cutting video frames, cutting each video frame into image blocks by means of windowing, and setting M image blocks for one frame;
[0056] Step S120, combining the trimmed image blocks, in the video sequence, randomly select N starting points, continuously take T fr...
PUM
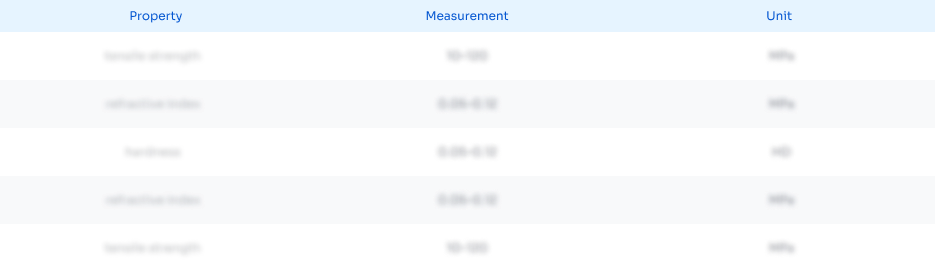
Abstract
Description
Claims
Application Information
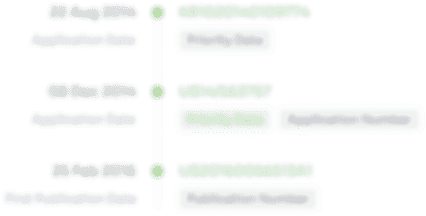
- R&D
- Intellectual Property
- Life Sciences
- Materials
- Tech Scout
- Unparalleled Data Quality
- Higher Quality Content
- 60% Fewer Hallucinations
Browse by: Latest US Patents, China's latest patents, Technical Efficacy Thesaurus, Application Domain, Technology Topic, Popular Technical Reports.
© 2025 PatSnap. All rights reserved.Legal|Privacy policy|Modern Slavery Act Transparency Statement|Sitemap|About US| Contact US: help@patsnap.com