Millimeter wave sensor gesture recognition method based on convolutional neural network
A technology of convolutional neural network and gesture recognition, which is applied in the field of gesture recognition based on convolutional neural network millimeter-wave sensors, can solve the problems of gesture recognition, such as limitations of lighting and climate conditions, short working distance, and low recognition accuracy, and achieve strong universal The effect of adaptability and adaptability, long-distance action, and avoidance of manual intervention
- Summary
- Abstract
- Description
- Claims
- Application Information
AI Technical Summary
Problems solved by technology
Method used
Image
Examples
Embodiment 1
[0032] Such as figure 1 , the method includes the following steps:
[0033] Step 1. Use the millimeter wave sensor to transmit frequency-modulated continuous wave signals, and perform various gestures in front of the sensor. The receiving channel of the millimeter wave sensor acquires time-domain echo signals of various types of gestures.
[0034] Step 2, performing zero-notch filtering and short-time Fourier transform on the acquired time-domain echo signal to obtain a micro-Doppler time-frequency map of finger motion;
[0035] (2.1) Carry out zero-notch filtering on the echo signal to filter out the stationary target signal. Use a notch filter to zero-filter the time-domain signal sequence x(n) of the gesture:
[0036]
[0037] Among them, y(n) is the output signal sequence; a i and b i is the filter coefficient; perform z-transformation on both sides of the formula (1), and obtain the transfer function of the digital filter as:
[0038]
[0039] Among them, z i ...
Embodiment 2
[0060] In the present invention, if image 3 As shown, a total of 12 time-frequency diagrams including coming, going, pressing down, bouncing, rotating, pushing, twisting fingers, reversing palms, sliding from left to right, sliding from right to left, zooming in, and zooming out are collected in total. Each gesture is collected 25 times, with a total of 300 time-frequency images, of which 5 time-frequency images of each type of test sample data, a total of 60 images. The original training sample data is 20 time-frequency images of each category, and the imagemagick development kit is used to sharpen each time-frequency image with a sharpening index of 10, and the image obtained after sharpening is the newly obtained training sample set; The brightness and saturation of the original training samples and the newly generated training samples after sharpening are adjusted to 80% of themselves, and a new batch of training samples is obtained; then all the above training samples ar...
PUM
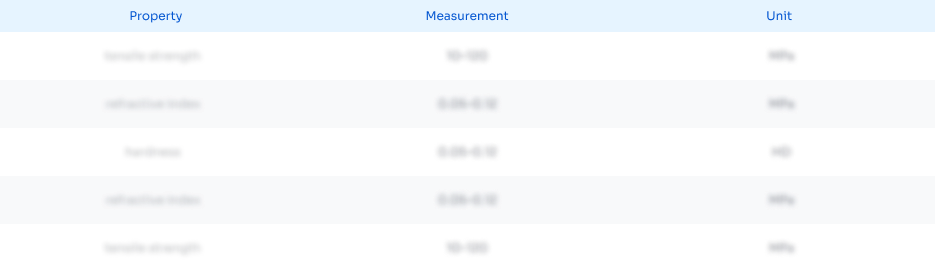
Abstract
Description
Claims
Application Information
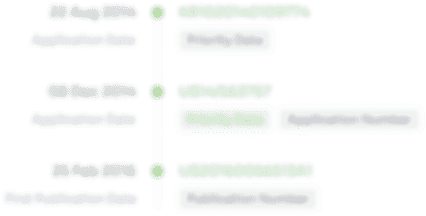
- R&D Engineer
- R&D Manager
- IP Professional
- Industry Leading Data Capabilities
- Powerful AI technology
- Patent DNA Extraction
Browse by: Latest US Patents, China's latest patents, Technical Efficacy Thesaurus, Application Domain, Technology Topic, Popular Technical Reports.
© 2024 PatSnap. All rights reserved.Legal|Privacy policy|Modern Slavery Act Transparency Statement|Sitemap|About US| Contact US: help@patsnap.com