Anti-collision control method based on depth reinforcement learning
A technology of reinforcement learning and control methods, applied in the field of assisted driving and automatic driving, can solve problems such as weak adaptive ability, high cost, and inability to adapt to early warning requirements, and achieve the effect of improving control performance and strong adaptability
- Summary
- Abstract
- Description
- Claims
- Application Information
AI Technical Summary
Problems solved by technology
Method used
Image
Examples
Embodiment Construction
[0030] In the drawings, the same or similar reference numerals are used to denote the same or similar elements or elements having the same or similar functions. Embodiments of the present invention will be described in detail below in conjunction with the accompanying drawings.
[0031]The anti-collision control method based on deep reinforcement learning provided in this embodiment adopts a deep deterministic policy gradient method for deep reinforcement learning, and the method includes the following steps:
[0032] Step 1, extract the vehicle parameters and environment vehicle parameters. in:
[0033] The own vehicle parameters include the speed v at which the own vehicle 1 is traveling.
[0034] Taking the three-lane situation as an example, the surrounding vehicles include the vehicles driving in the same lane as the own vehicle 1 and longitudinally in front of the own vehicle 1 (hereinafter referred to as "the front vehicle 2"), and the vehicles driving in the lane whe...
PUM
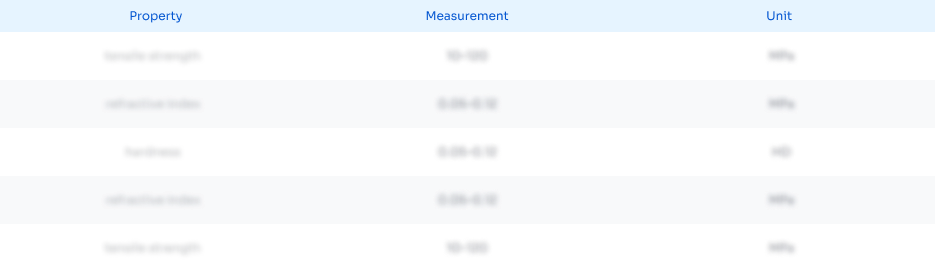
Abstract
Description
Claims
Application Information
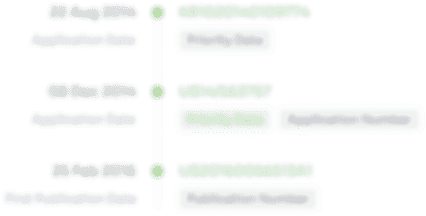
- R&D
- Intellectual Property
- Life Sciences
- Materials
- Tech Scout
- Unparalleled Data Quality
- Higher Quality Content
- 60% Fewer Hallucinations
Browse by: Latest US Patents, China's latest patents, Technical Efficacy Thesaurus, Application Domain, Technology Topic, Popular Technical Reports.
© 2025 PatSnap. All rights reserved.Legal|Privacy policy|Modern Slavery Act Transparency Statement|Sitemap|About US| Contact US: help@patsnap.com