A method for EEG classification based on frequency-band attention residual network
A classification method and attention technology, applied in biological neural network models, medical science, diagnosis, etc., can solve problems such as lack of flexibility, and achieve the effect of obtaining flexibility
- Summary
- Abstract
- Description
- Claims
- Application Information
AI Technical Summary
Problems solved by technology
Method used
Image
Examples
Embodiment Construction
[0016] The present invention will be further described below in conjunction with the accompanying drawings and specific embodiments.
[0017] The flow process of the method involved in the present invention comprises the following steps:
[0018] (1) EEG signal preprocessing.
[0019] Use EEGLab to perform baseline removal, band-pass filtering, independent component analysis and artifact removal operations on the original N-lead EEG signals with a total duration of T seconds and a sampling frequency of M. The range of band-pass filtering is between 0.5 Hz and 47 Hz. between.
[0020] (2) EEG signal segmentation.
[0021] Use a sliding window with a segment length of W seconds to segment the N-lead EEG signals processed in (1), there is no overlap between segments, and a total of S data segments are obtained, and each data segment has a dimension of A two-dimensional matrix of N*(W*M), where S is the result of dividing the original data duration T by the sliding window segme...
PUM
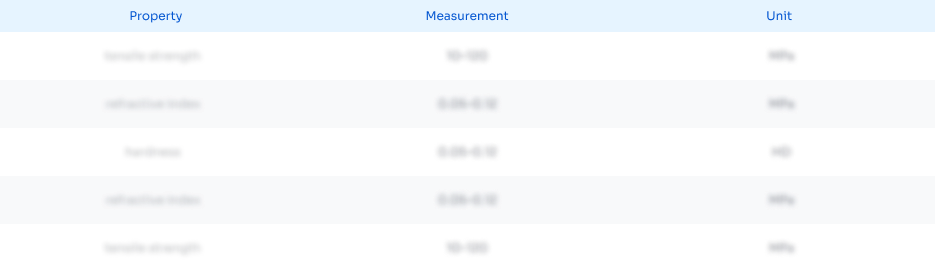
Abstract
Description
Claims
Application Information
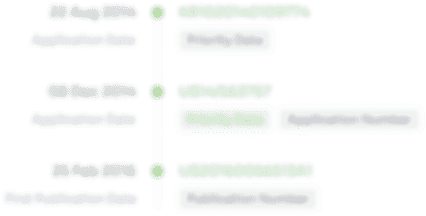
- Generate Ideas
- Intellectual Property
- Life Sciences
- Materials
- Tech Scout
- Unparalleled Data Quality
- Higher Quality Content
- 60% Fewer Hallucinations
Browse by: Latest US Patents, China's latest patents, Technical Efficacy Thesaurus, Application Domain, Technology Topic, Popular Technical Reports.
© 2025 PatSnap. All rights reserved.Legal|Privacy policy|Modern Slavery Act Transparency Statement|Sitemap|About US| Contact US: help@patsnap.com