Model training method for cross-domain sentiment analysis based on convolutional neural network
A technology of convolutional neural network and sentiment analysis, which is applied in the field of cross-domain sentiment analysis model training, can solve problems such as poor classification effect, and achieve the effect of reducing labeling
- Summary
- Abstract
- Description
- Claims
- Application Information
AI Technical Summary
Problems solved by technology
Method used
Image
Examples
Embodiment
[0061] 1.1 Solve the problem
[0062] The present invention proposes a method based on neural network model migration to solve the problem of cross-domain sentiment analysis. Commonly used sentiment classification research methods include processing Chinese and English corpus, data vector representation, feature extraction, and classification. Explain the method and use of the data preprocessing model, and introduce the relevant mathematical principles and framework of Word2vec, verify that the use of depth migration method can effectively solve the learning effect on different tasks, the deep network framework used in the present invention is in CNN convolution Transfer based on the neural network model. Model migration is performed on cross-domain emotional texts, and fine-truning is performed on existing models in the target domain to solve the problem of cross-domain sentiment analysis.
[0063] 2.1 Sentiment Analysis
[0064] 2.1.1 Basic concepts
[0065] Text sentimen...
PUM
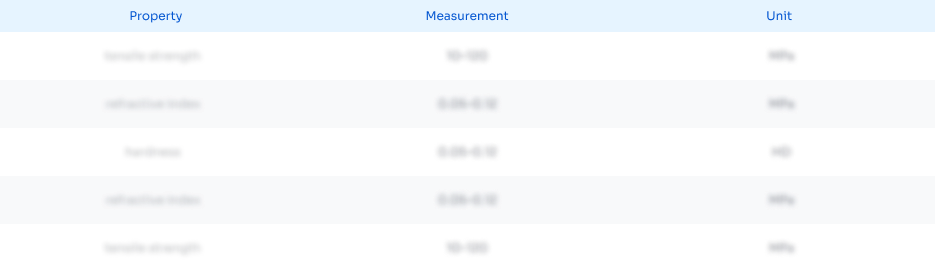
Abstract
Description
Claims
Application Information
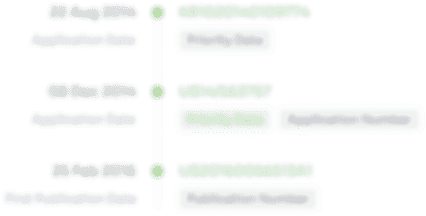
- R&D Engineer
- R&D Manager
- IP Professional
- Industry Leading Data Capabilities
- Powerful AI technology
- Patent DNA Extraction
Browse by: Latest US Patents, China's latest patents, Technical Efficacy Thesaurus, Application Domain, Technology Topic, Popular Technical Reports.
© 2024 PatSnap. All rights reserved.Legal|Privacy policy|Modern Slavery Act Transparency Statement|Sitemap|About US| Contact US: help@patsnap.com