Reinforcement learning model optimization method and device based on parameter noise
A technology of reinforcement learning and optimization methods, applied in the field of reinforcement learning model optimization based on parameter noise, can solve problems such as low recognition accuracy and slow convergence speed, and achieve the effects of accelerating model convergence, accelerating convergence speed, and improving exploration efficiency.
- Summary
- Abstract
- Description
- Claims
- Application Information
AI Technical Summary
Problems solved by technology
Method used
Image
Examples
Embodiment Construction
[0029] The present invention will be further described below in conjunction with the accompanying drawings and preferred embodiments.
[0030] It has been introduced above that the present invention can be widely used in unmanned driving, mechanical arm control and other aspects in the industry. However, for the convenience of verification, the model effect of a reinforcement learning model optimization method based on parameter noise proposed in this embodiment is verified on a variety of game simulators. The game simulator website can be found at http: / / www.mujoco.org / , the simulation results show that this embodiment has achieved better results than previous ones. The reason why the game simulator is used for verification is that the verification cost is low, variables are easy to control, and the verification effect is better. The simulator verification is actually a reliability evaluation of the actual system construction.
[0031] The estimation method includes the fo...
PUM
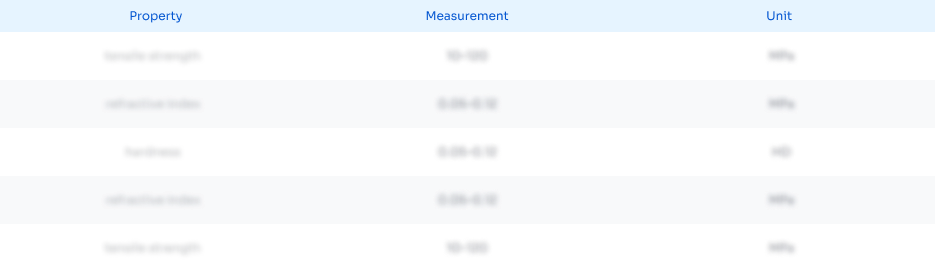
Abstract
Description
Claims
Application Information
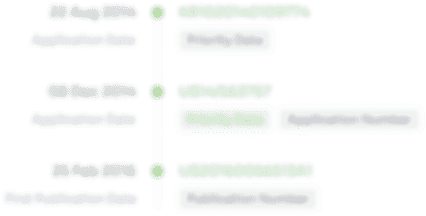
- R&D Engineer
- R&D Manager
- IP Professional
- Industry Leading Data Capabilities
- Powerful AI technology
- Patent DNA Extraction
Browse by: Latest US Patents, China's latest patents, Technical Efficacy Thesaurus, Application Domain, Technology Topic, Popular Technical Reports.
© 2024 PatSnap. All rights reserved.Legal|Privacy policy|Modern Slavery Act Transparency Statement|Sitemap|About US| Contact US: help@patsnap.com