Hyperspectral Image Classification Method and System Based on Correlation Entropy Principle
A technology of hyperspectral images and classification methods, applied in character and pattern recognition, instruments, computing, etc., can solve the problems of difficulty in learning effective classification features, high sample complexity, and achieve the effect of small sample complexity
- Summary
- Abstract
- Description
- Claims
- Application Information
AI Technical Summary
Problems solved by technology
Method used
Image
Examples
Embodiment Construction
[0050] The technical solutions and effects of the present invention will be described in further detail below with reference to the accompanying drawings.
[0051] refer to figure 1 , the implementation steps of the present invention are as follows:
[0052] 1) Input a hyperspectral image, and normalize the image so that the range is within [0, 1]. Order I tr ={I 1 ,I 2 ,Λ,I N} is a training set consisting of N pixels, where I i ∈ R d (i=1, 2, Λ, N) is the i-th training sample, and they belong to class C; normalize the image, and normalize the data value to [0, 1] by the following steps:
[0053]
[0054] Among them, M x =max(I(:)),M n =min(I(:)) are the maximum and minimum values of pixel values on the input image, respectively, and is the pixel I with coordinates (i,j) ij B bands, is the pixel with coordinates (i, j) on the normalized hyperspectral image B bands.
[0055] 2) Select p% of the pixels from the hyperspectral image as training samples,...
PUM
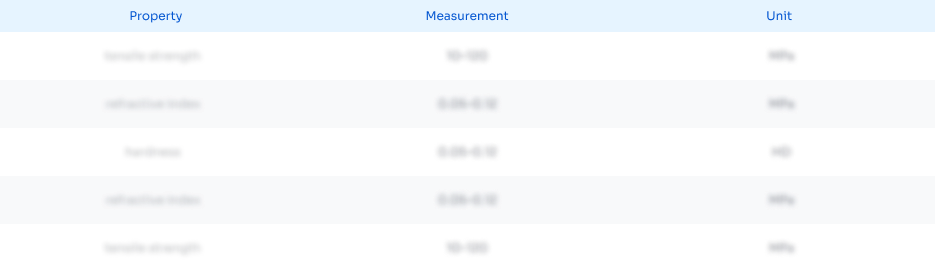
Abstract
Description
Claims
Application Information
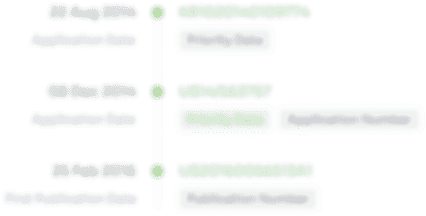
- Generate Ideas
- Intellectual Property
- Life Sciences
- Materials
- Tech Scout
- Unparalleled Data Quality
- Higher Quality Content
- 60% Fewer Hallucinations
Browse by: Latest US Patents, China's latest patents, Technical Efficacy Thesaurus, Application Domain, Technology Topic, Popular Technical Reports.
© 2025 PatSnap. All rights reserved.Legal|Privacy policy|Modern Slavery Act Transparency Statement|Sitemap|About US| Contact US: help@patsnap.com