Coal equipment fault early warning system and method based on machine learning
A technology of equipment failure and machine learning, applied in database management systems, instruments, special data processing applications, etc., can solve problems such as impact, inaccurate alarms, and inability to guarantee historical data status data
- Summary
- Abstract
- Description
- Claims
- Application Information
AI Technical Summary
Problems solved by technology
Method used
Image
Examples
Example Embodiment
[0089] The present invention will be further described below in conjunction with the accompanying drawings and embodiments.
[0090] The present invention proposes a coal equipment failure early warning system and method based on machine learning by considering on-site environmental factors, equipment operating condition types and adopting various discrimination rules, thereby improving the real-time and accuracy of coal equipment fault early warning.
[0091] like figure 1 As shown, the coal equipment failure warning system based on machine learning of the present invention includes a data signal acquisition unit, a database, a machine learning platform, a machine learning algorithm module and a result display unit;
[0092]Data signal acquisition unit: used to collect real-time data and obtain static data;
[0093] Database: obtain real-time data and static data from the data signal acquisition unit, organize the real-time data and static data according to the hierarchical ...
PUM
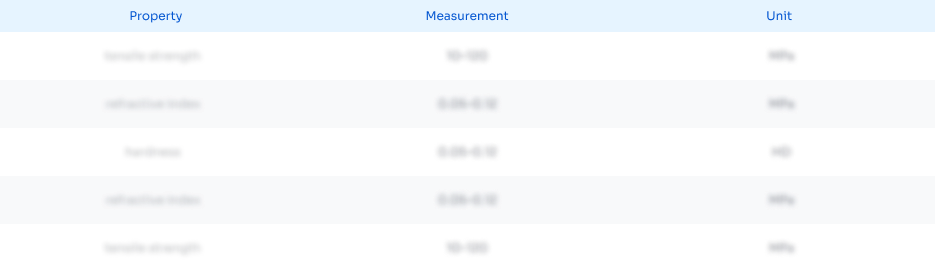
Abstract
Description
Claims
Application Information
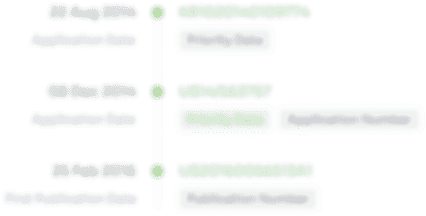
- R&D Engineer
- R&D Manager
- IP Professional
- Industry Leading Data Capabilities
- Powerful AI technology
- Patent DNA Extraction
Browse by: Latest US Patents, China's latest patents, Technical Efficacy Thesaurus, Application Domain, Technology Topic, Popular Technical Reports.
© 2024 PatSnap. All rights reserved.Legal|Privacy policy|Modern Slavery Act Transparency Statement|Sitemap|About US| Contact US: help@patsnap.com