Mobile pollution source emission concentration prediction method based on fuzzy weighting ELM
A prediction method and emission concentration technology, applied in neural learning methods, biological neural network models, special data processing applications, etc., can solve the problems of low accuracy and achieve the effects of improving real-time performance, reducing computational complexity, and high prediction accuracy
- Summary
- Abstract
- Description
- Claims
- Application Information
AI Technical Summary
Benefits of technology
Problems solved by technology
Method used
Image
Examples
Embodiment Construction
[0047] like figure 1 Shown, the present invention is concretely realized as follows:
[0048]Step 1: Initialize training samples. The training set and the test set are normalized respectively by using the maximum and minimum method, and converted into a value between [0,1]; % to divide the training set and test set.
[0049] Step 2: Select the Gaussian membership function parameters and calculate the degree of membership of each input variable The specific method is as follows:
[0050] The Takagi-Sugeno-Kang (TSK) fuzzy system can learn and memorize temporal information implicitly. The TSK fuzzy system is defined by the following "if-then" rule form, where the rule is R i In the case of , the fuzzy reasoning is as follows:
[0051] Rule R t :
[0052] in, is the fuzzy set of the fuzzy system; (j=1, 2,...k) is a fuzzy system parameter; y i For the output obtained according to the fuzzy rules, the input part (ie the if part) is fuzzy, and the output part (ie the...
PUM
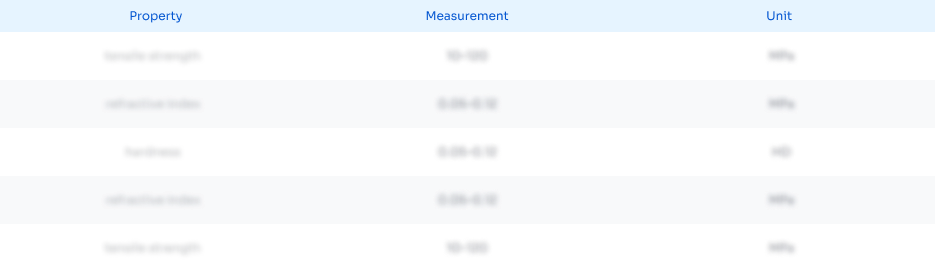
Abstract
Description
Claims
Application Information
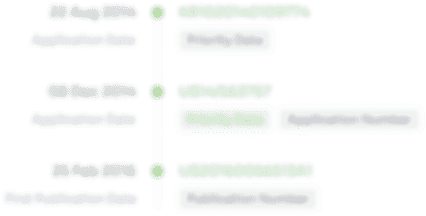
- R&D Engineer
- R&D Manager
- IP Professional
- Industry Leading Data Capabilities
- Powerful AI technology
- Patent DNA Extraction
Browse by: Latest US Patents, China's latest patents, Technical Efficacy Thesaurus, Application Domain, Technology Topic, Popular Technical Reports.
© 2024 PatSnap. All rights reserved.Legal|Privacy policy|Modern Slavery Act Transparency Statement|Sitemap|About US| Contact US: help@patsnap.com