Visual data completion method based on local low-rank tensor estimation
A data and visual technology, applied in the field of visual data completion, can solve the problems of missing tensor interpolation, ignoring the characteristics of local high correlation, and high computational complexity, and achieve the effect of high correlation
- Summary
- Abstract
- Description
- Claims
- Application Information
AI Technical Summary
Problems solved by technology
Method used
Image
Examples
Embodiment
[0031] The specific implementation steps of the visual data completion method based on local low-rank tensor estimation in the present invention include:
[0032] (1) Obtain visual data and store it as tensor data with missing values Take the target tensor Ω is the coordinate set of non-missing value data.
[0033] (2) the target tensor decomposes into n with overlap g sub tensor and have Ω i Corresponding to the coordinates of extracting subtensor elements by row and column height, such as figure 1 As shown, the picture shows the process of decomposing the tensor data with missing values into sub-tensors with overlapping, in which the target tensor is decomposed into n subtensors The elements contained in these sub-tensors overlap each other. This method performs matrix expansion on each sub-tensor and performs low-rank estimation to solve the target tensor The missing element value in , the specific steps are:
[0034] 21) Determine the size of the subtensor...
PUM
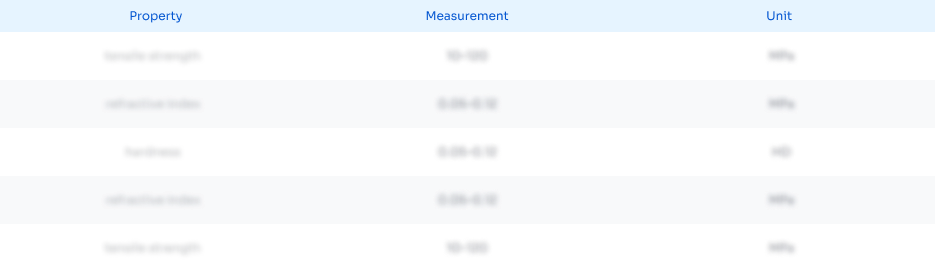
Abstract
Description
Claims
Application Information
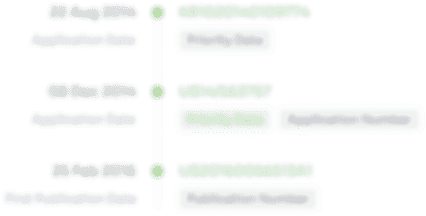
- R&D
- Intellectual Property
- Life Sciences
- Materials
- Tech Scout
- Unparalleled Data Quality
- Higher Quality Content
- 60% Fewer Hallucinations
Browse by: Latest US Patents, China's latest patents, Technical Efficacy Thesaurus, Application Domain, Technology Topic, Popular Technical Reports.
© 2025 PatSnap. All rights reserved.Legal|Privacy policy|Modern Slavery Act Transparency Statement|Sitemap|About US| Contact US: help@patsnap.com