Training Sample Selection Method Based on Multi-Instance Learning Idea in Target Tracking
A multi-instance learning and target tracking technology, which is applied in image analysis, image enhancement, instruments, etc., can solve the problem that the sample label cannot be guaranteed
- Summary
- Abstract
- Description
- Claims
- Application Information
AI Technical Summary
Problems solved by technology
Method used
Image
Examples
specific Embodiment approach 1
[0042] DETAILED DESCRIPTION 1. A method for selecting training samples based on the idea of multi-instance learning in target tracking. The present invention proposes a method for selecting training samples based on the idea of multiple instance learning (MIL) for target tracking. The main idea is to put all the positive samples into a positive sample package, and consider the samples that contribute less to the log-likelihood function of the package as the poor samples. In an iterative way, the worst samples are removed from the positive sample packet in each iteration until a sufficient number of samples remain in the positive sample packet.
[0043] The specific steps of the invention are:
[0044] Input: training set {(X 1 , Y 1 ),..., (X n , Y n )}, where X 1 ={x 11 ,..., x 1M } Is the first sample package, which is also a positive sample package, {X 2 ,..., X n } Is a negative sample package, y i ∈{0,1} is the label of the packet;
[0045] 1: Use the data set {(X 2 , Y 2 )...
PUM
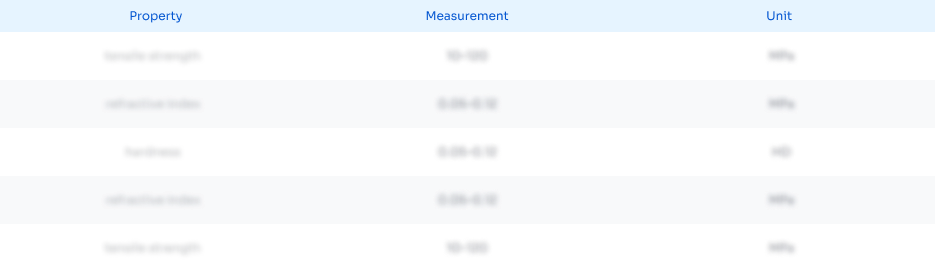
Abstract
Description
Claims
Application Information
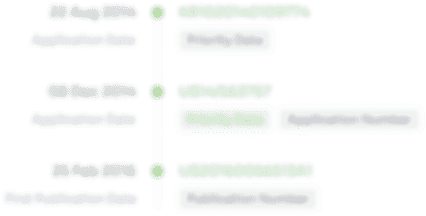
- Generate Ideas
- Intellectual Property
- Life Sciences
- Materials
- Tech Scout
- Unparalleled Data Quality
- Higher Quality Content
- 60% Fewer Hallucinations
Browse by: Latest US Patents, China's latest patents, Technical Efficacy Thesaurus, Application Domain, Technology Topic, Popular Technical Reports.
© 2025 PatSnap. All rights reserved.Legal|Privacy policy|Modern Slavery Act Transparency Statement|Sitemap|About US| Contact US: help@patsnap.com