DCNN (Deep Convolutional Neural Network) based 3D shape classification method
A convolutional neural network and neural network technology, applied in the field of three-dimensional shape classification based on deep convolutional neural network, can solve the problems of small three-dimensional shape data set and time-consuming images.
- Summary
- Abstract
- Description
- Claims
- Application Information
AI Technical Summary
Problems solved by technology
Method used
Image
Examples
Embodiment Construction
[0032] It should be noted that, in the case of no conflict, the embodiments in the present application and the features in the embodiments can be combined with each other. The present invention will be further described in detail below in conjunction with the drawings and specific embodiments.
[0033] figure 1 It is a system flowchart of a three-dimensional shape classification method based on a deep convolutional neural network in the present invention. It mainly includes data input; initial convolutional neural network; beam search; knowledge transfer.
[0034] Wherein, the data input uses a three-dimensional entity set as a data set, which includes 40 various types of physical object classes, such as chairs, tables, toilets, sofas, etc.; each class has 100 unique CAD models, representing The most common 3D shapes, there are a total of 151,128 voxelized models in the entire dataset.
[0035] Wherein, the initial convolutional neural network is a relatively simple initial ...
PUM
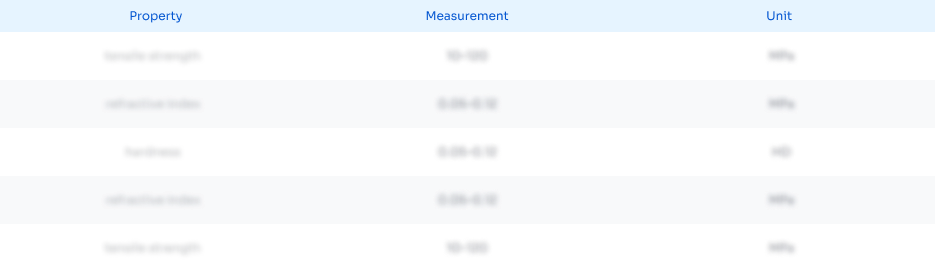
Abstract
Description
Claims
Application Information
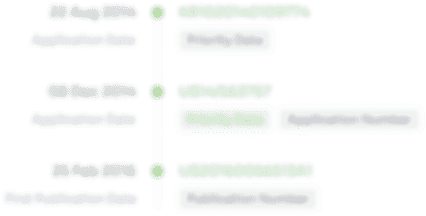
- R&D Engineer
- R&D Manager
- IP Professional
- Industry Leading Data Capabilities
- Powerful AI technology
- Patent DNA Extraction
Browse by: Latest US Patents, China's latest patents, Technical Efficacy Thesaurus, Application Domain, Technology Topic.
© 2024 PatSnap. All rights reserved.Legal|Privacy policy|Modern Slavery Act Transparency Statement|Sitemap