Matrix decomposition recommendation method in graph construction framework
A technology of matrix decomposition and recommendation method, applied in the field of recommendation learning, to achieve the effect of improving recommendation accuracy, fast convergence, and improving performance
- Summary
- Abstract
- Description
- Claims
- Application Information
AI Technical Summary
Problems solved by technology
Method used
Image
Examples
Embodiment Construction
[0036] Such as figure 1 As shown, this embodiment discloses a matrix decomposition recommendation method under the graph construction framework, including the following steps:
[0037] Step 1: Create a rating matrix based on user rating data. The scoring matrix R is specifically described in Table 1.
[0038] Step 2: Add constraint items to the score matrix decomposition model based on manifold regularization to update the similarity information, so as to establish a new decomposition model as:
[0039]
[0040] In the formula, m represents the number of users, and n represents the number of items. If user u has rated item i, then I ui Denoted as 1, if user u does not rate item i, then I ui represented as 0, r ui Represents the rating value of user u on item i, μ represents the global average of all recorded ratings, b u Denotes the user bias vector of user u, b i Denotes the item bias vector of item i, p u ,q i is the latent semantic feature vector, p u Represents...
PUM
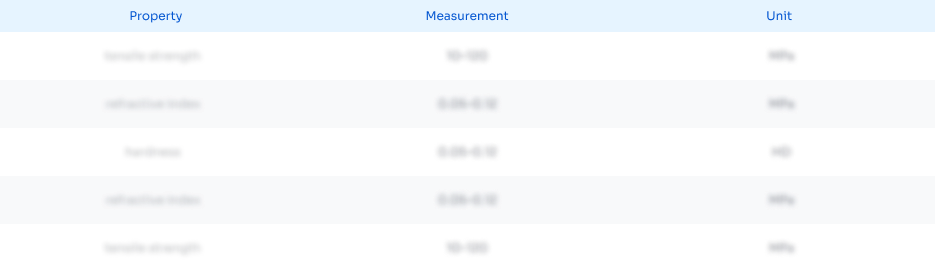
Abstract
Description
Claims
Application Information
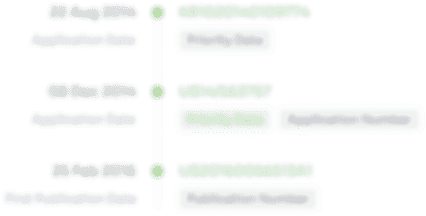
- R&D Engineer
- R&D Manager
- IP Professional
- Industry Leading Data Capabilities
- Powerful AI technology
- Patent DNA Extraction
Browse by: Latest US Patents, China's latest patents, Technical Efficacy Thesaurus, Application Domain, Technology Topic, Popular Technical Reports.
© 2024 PatSnap. All rights reserved.Legal|Privacy policy|Modern Slavery Act Transparency Statement|Sitemap|About US| Contact US: help@patsnap.com