Hyper-spectral image classification method based on local manifold embedding
A technology of hyperspectral image and classification method, which is applied in the field of hyperspectral image classification based on local manifold embedding, and can solve problems such as the inherent manifold that cannot effectively characterize the data.
- Summary
- Abstract
- Description
- Claims
- Application Information
AI Technical Summary
Problems solved by technology
Method used
Image
Examples
Embodiment Construction
[0094] According to the process of the MFA algorithm, it only considers the neighborhood structure of the data when composing the image. For hyperspectral images with a large number of homogeneous regions, MFA cannot effectively characterize the internal manifold of the data. In order to improve the effect of MFA algorithm in feature extraction of hyperspectral images, the present invention proposes a new manifold learning method called Local Manifold Embedding (LME).
[0095] The present invention uses the neighborhood of data and the neighborhood of each neighborhood point to characterize the intrinsic structure of the hyperspectral image. First, each data point is reconstructed using the same kind of neighbor points, and then, the neighborhood of each data point and the reconstruction points corresponding to each neighborhood point are used to construct the intra-class graph, intra-class reconstruction graph, inter-class graph and class graph. Finally, in the low-dimensiona...
PUM
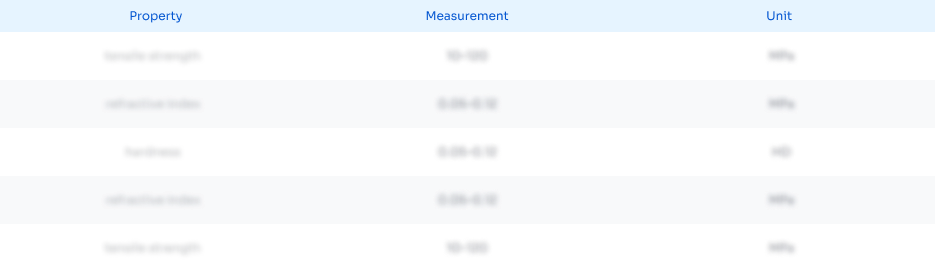
Abstract
Description
Claims
Application Information
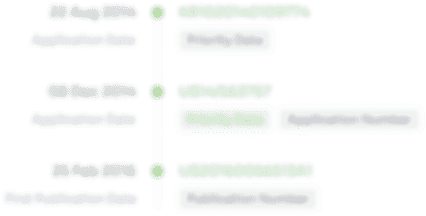
- R&D Engineer
- R&D Manager
- IP Professional
- Industry Leading Data Capabilities
- Powerful AI technology
- Patent DNA Extraction
Browse by: Latest US Patents, China's latest patents, Technical Efficacy Thesaurus, Application Domain, Technology Topic, Popular Technical Reports.
© 2024 PatSnap. All rights reserved.Legal|Privacy policy|Modern Slavery Act Transparency Statement|Sitemap|About US| Contact US: help@patsnap.com