A multi-kernel learning method for hyperspectral image classification that maximizes class separability
A hyperspectral image and multi-core learning technology, which is applied in character and pattern recognition, instruments, calculations, etc., can solve problems such as low solution efficiency and does not consider multi-core model solving and subsequent classification applications, so as to achieve high algorithm efficiency and improve algorithm operations Efficiency, the effect of improving classification performance
- Summary
- Abstract
- Description
- Claims
- Application Information
AI Technical Summary
Problems solved by technology
Method used
Image
Examples
specific Embodiment approach 1
[0017] Specific implementation mode 1. Combination figure 1 To illustrate this embodiment, a multi-core learning method based on maximizing category separability criterion described in this embodiment is performed in the following steps:
[0018] Step 1. Obtain training samples and test samples from a given input hyperspectral image dataset;
[0019] Step 2. Use the training sample set Construct the base kernel matrix K in the multi-kernel learning model m , get the base kernel matrix set
[0020] Step 3, using the base kernel matrix set to measure the intra-class dispersion and inter-class dispersion of the hyperspectral image dataset in the Hilbert kernel space;
[0021] Step 4: On the basis of intra-class dispersion and inter-class dispersion, class separability is measured with the maximum class interval criterion, and the maximum class separability is used as the solution criterion for the multi-kernel learning model to solve the base kernel weight.
[0022] This e...
specific Embodiment approach 2
[0025] Specific embodiment 2: The difference between this embodiment and specific embodiment 1 is that in the step 1, training samples and test samples are obtained from a given input hyperspectral image data set; the specific process is:
[0026] Step 11. The given input hyperspectral image dataset is: x i ∈ R F
[0027] Among them, T represents the total number of samples in the input hyperspectral image dataset, Input hyperspectral image dataset X input Contains samples of class C, and the number of samples of class l is N l ;1≤l≤C;x i For the i-th sample in the input hyperspectral image dataset, R F is a space representing dimension F, F is the feature dimension of the input hyperspectral image data set, and i is a positive integer;
[0028] Step 12. From the input hyperspectral image dataset X input Randomly select samples according to p% of each class to form a training sample set:
[0029] Among them, p is determined by the complexity of the input data and ...
specific Embodiment approach 3
[0031] Specific embodiment three: the difference between this embodiment and specific embodiment one or two is that the training sample set is used in the second step Construct the base kernel matrix K in the multi-kernel learning model m , get the base kernel matrix set The base kernel matrix is constructed as follows:
[0032] The training sample set The middle samples are input in pairs to the Gaussian kernel function to calculate the base kernel matrix where σ m is the Gaussian kernel scale parameter, x j is the jth sample in the training sample set, and i and j are positive integers.
[0033] Other steps and parameters are the same as those in Embodiment 1 or Embodiment 2.
PUM
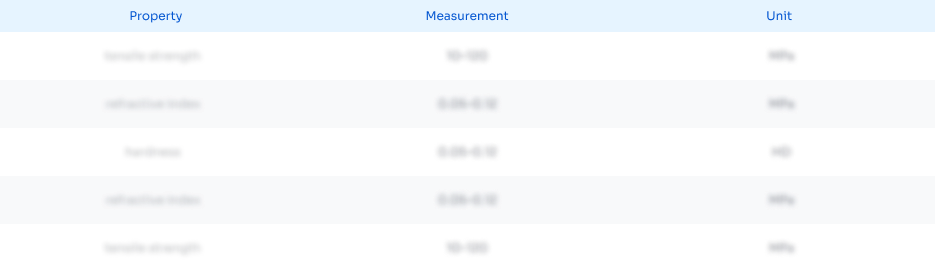
Abstract
Description
Claims
Application Information
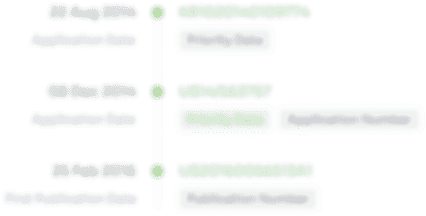
- R&D Engineer
- R&D Manager
- IP Professional
- Industry Leading Data Capabilities
- Powerful AI technology
- Patent DNA Extraction
Browse by: Latest US Patents, China's latest patents, Technical Efficacy Thesaurus, Application Domain, Technology Topic, Popular Technical Reports.
© 2024 PatSnap. All rights reserved.Legal|Privacy policy|Modern Slavery Act Transparency Statement|Sitemap|About US| Contact US: help@patsnap.com