Weighted K-means method for grouping of power customer values
A customer group and power technology, applied in the direction of instruments, character and pattern recognition, data processing applications, etc., can solve the problems of large differences in distribution density, high-density small groups carve up low-density large groups, etc., to achieve high returns and improve clustering compactness sexual effect
- Summary
- Abstract
- Description
- Claims
- Application Information
AI Technical Summary
Problems solved by technology
Method used
Image
Examples
Embodiment Construction
[0019] The present invention will be further described in detail below in conjunction with the accompanying drawings and embodiments.
[0020] The weighted K-means clustering method process of power customer value grouping, the specific steps are as follows:
[0021] Step 1: Data preprocessing. Firstly, exploratory analysis is carried out on the original power customer marketing data. On this basis, the variables irrelevant to the analysis target are eliminated or the variables required by the model are extracted (constructed), and these selected data are processed. Through power customer marketing data cleaning, data integration and data transformation, the original power customer marketing data is processed into the input feature data set required by the model.
[0022] Step 2: Initial clustering of the power customer characteristic variable data set that has been processed in step 1. First, use random method to select K initial clustering centers, and use the shortest Euc...
PUM
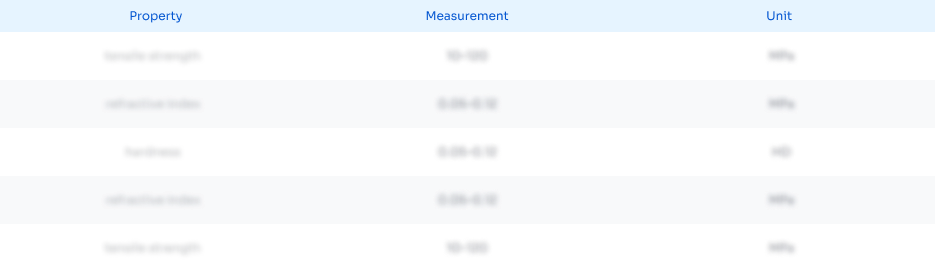
Abstract
Description
Claims
Application Information
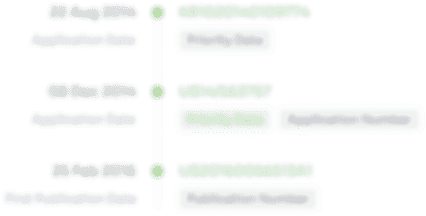
- R&D Engineer
- R&D Manager
- IP Professional
- Industry Leading Data Capabilities
- Powerful AI technology
- Patent DNA Extraction
Browse by: Latest US Patents, China's latest patents, Technical Efficacy Thesaurus, Application Domain, Technology Topic, Popular Technical Reports.
© 2024 PatSnap. All rights reserved.Legal|Privacy policy|Modern Slavery Act Transparency Statement|Sitemap|About US| Contact US: help@patsnap.com