Active learning big data mark method and system
An active learning, big data technology, applied in the field of big machine learning, can solve the problem of low accuracy of big data anchor point labeling
- Summary
- Abstract
- Description
- Claims
- Application Information
AI Technical Summary
Problems solved by technology
Method used
Image
Examples
Embodiment approach
[0055] As a specific implementation, the active learning big data labeling method also includes the following steps:
[0056] Use the kernel matrix K to perform nonlinear mapping on the data points, and obtain the distance after nonlinear mapping
[0057] Using a greedy sequential method, the anchor data set used for active learning is determined according to the following formula:
[0058] z t ∈X and
[0059] Among them, Z t-1 ={z 1 ,…,z t-1} is assumed to have determined t-1 anchor points, z i =x p(i) , p represents the subscript correspondence, Indicates that the t-th anchor point is determined according to the formula,
[0060]
[0061] Initialize Z=φ, according to t=1,...,m sequentially calculate coefficient, keep unchanged, calculated as well as Update according to the proximal point method Sure to make get the minimum yes, and where Tr(·) represents the trace of the matrix, represents the pth of the kernel matrix K i Row.
[006...
PUM
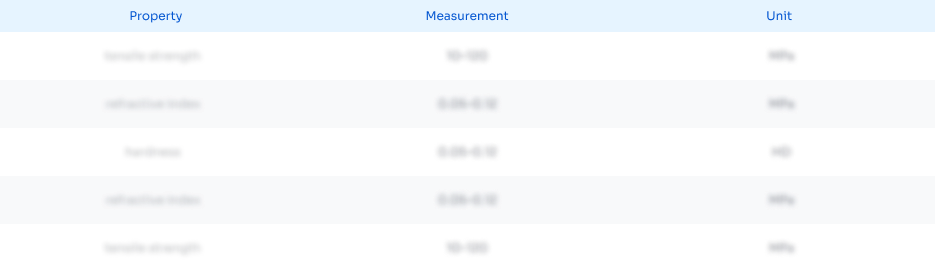
Abstract
Description
Claims
Application Information
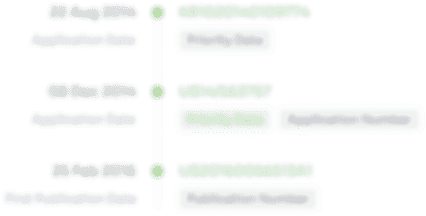
- R&D Engineer
- R&D Manager
- IP Professional
- Industry Leading Data Capabilities
- Powerful AI technology
- Patent DNA Extraction
Browse by: Latest US Patents, China's latest patents, Technical Efficacy Thesaurus, Application Domain, Technology Topic, Popular Technical Reports.
© 2024 PatSnap. All rights reserved.Legal|Privacy policy|Modern Slavery Act Transparency Statement|Sitemap|About US| Contact US: help@patsnap.com