Improved sift face feature extraction method based on key points
A face feature and extraction method technology, applied in the field of face recognition, can solve the problem of not being able to accurately locate the key points of the face, and achieve the effect of reducing the number of dimensions
- Summary
- Abstract
- Description
- Claims
- Application Information
AI Technical Summary
Problems solved by technology
Method used
Image
Examples
Embodiment Construction
[0055] The basic flow of the improved SIFT face feature extraction method based on key points of the present invention is as follows: figure 1 As shown, it specifically includes the following steps:
[0056] 1) Divide the data in the face database into 10 groups for cross-validation experiments, of which 9 groups of data are used as training data, and the remaining 1 group of data is used as test data. Each group contains 300 pairs of face pictures from the same person and 300 pairs of Pictures of faces from different people. For each face picture, use a three-layer deep convolutional network cascade to locate the coordinate positions of five key pixels (the pixel in the middle of the left eye, the pixel in the middle of the right eye, the pixel on the tip of the nose, the corner of the left mouth pixels and pixels at the corner of the right mouth).
[0057] In the first layer of the three-layer deep convolutional network cascade structure, three deep convolutional networks ...
PUM
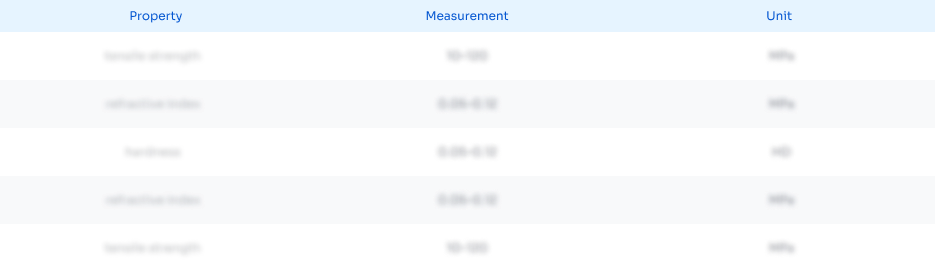
Abstract
Description
Claims
Application Information
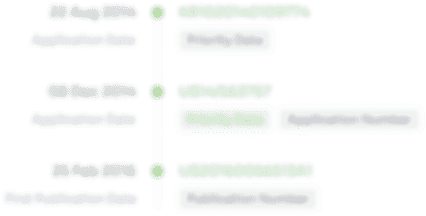
- R&D Engineer
- R&D Manager
- IP Professional
- Industry Leading Data Capabilities
- Powerful AI technology
- Patent DNA Extraction
Browse by: Latest US Patents, China's latest patents, Technical Efficacy Thesaurus, Application Domain, Technology Topic, Popular Technical Reports.
© 2024 PatSnap. All rights reserved.Legal|Privacy policy|Modern Slavery Act Transparency Statement|Sitemap|About US| Contact US: help@patsnap.com