Integrated multi-objective evolutionary automatic clustering method based on minimum spinning tree
A multi-objective evolution and automatic clustering technology, which is applied in the field of integrated multi-objective evolutionary automatic clustering, can solve the problems of reducing accuracy, reducing the classification accuracy of gene expression data, increasing the burden of classifier design, etc., and achieving enhanced search capabilities Effect
- Summary
- Abstract
- Description
- Claims
- Application Information
AI Technical Summary
Benefits of technology
Problems solved by technology
Method used
Image
Examples
Embodiment Construction
[0048] Combine below figure 1 The specific implementation steps of the present invention are further described in detail.
[0049] Step 1. Input the gene dataset to be clustered.
[0050] Step 2. Initialize.
[0051] When c>2, the category number interval of the initial population individual is [c-2,c+2], when c≤2, the category number interval of the initial population individual is [2,c+2], where c means The true number of categories of the gene dataset to be clustered.
[0052] Using the K-means algorithm, each value in the category number interval of the gene data set to be clustered is used as the number of categories of the gene data set to be clustered, and the gene data set to be clustered with the determined number of categories is clustered to obtain different The K-means base clustering population;
[0053] Using the average distance algorithm, each value in the category number interval of the gene data set to be clustered is used as the number of categories of t...
PUM
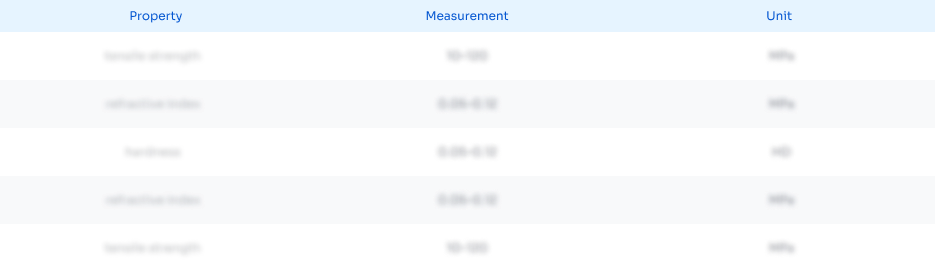
Abstract
Description
Claims
Application Information
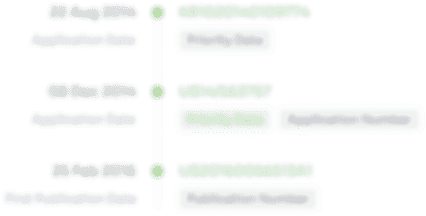
- R&D Engineer
- R&D Manager
- IP Professional
- Industry Leading Data Capabilities
- Powerful AI technology
- Patent DNA Extraction
Browse by: Latest US Patents, China's latest patents, Technical Efficacy Thesaurus, Application Domain, Technology Topic, Popular Technical Reports.
© 2024 PatSnap. All rights reserved.Legal|Privacy policy|Modern Slavery Act Transparency Statement|Sitemap|About US| Contact US: help@patsnap.com