Feature selection based multi-example multi-tag learning method and system
A technology of feature selection and learning method, applied in the field of pattern recognition and machine learning, can solve the problems of being susceptible to noise, loss of label related information, sensitive to the number of classifier clusters, etc., to improve the labeling effect, increase the available information, The effect of improving accuracy
- Summary
- Abstract
- Description
- Claims
- Application Information
AI Technical Summary
Problems solved by technology
Method used
Image
Examples
Embodiment 1
[0025] Embodiment 1. A multi-instance multi-label learning method based on feature selection. Combine below figure 1 The method provided in this embodiment will be described in detail.
[0026] see figure 1 , S1. Map all package features in the multi-instance and multi-label known data set to the projected example reference space composed of all examples in the known data set, and obtain the feature vector of the package.
[0027] Specifically, the multi-instance and multi-label known data set specifically includes: the number of packages and packages in the known data set, the known examples and the number of examples in each package, the known feature vector representing each example, The label set corresponding to each package and the label set corresponding to all packages are known, wherein the known multi-instance multi-label data set is specifically expressed as {(X 1 ,Y 1 ),(X 2 ,Y 2 ),…,(X i ,Y i ),…,(X m ,Y m )},in, Package X for known datasets i n in i...
Embodiment 2
[0101] Embodiment 2. A method of predicting the label set of multi-instance and multi-label unknown samples using a multi-instance and multi-label learning method based on feature selection. Combine below figure 2 The method provided in this embodiment will be described in detail.
[0102] see figure 2 , this embodiment provides a method for predicting the label set of multi-instance and multi-label unknown samples by using a multi-instance multi-label learning method based on feature selection.
[0103] S51. Map all unknown package features in the multi-instance and multi-label unknown samples to be predicted to the representative projection example set In the reference space α of the representative projection example composed of , and then obtain the feature set of all unknown packages, where the unknown package P i After feature mapping, the q-dimensional feature vector ψ(P i ).
[0104] Specifically, the unknown packet P in the multi-instance multi-label unknown sa...
Embodiment 3
[0118] Embodiment 3, a multi-instance multi-label learning system based on feature selection. Combine below image 3 The system provided in this embodiment will be described in detail.
[0119] see image 3 A multi-instance multi-label learning system based on feature selection provided in this embodiment includes a known data set storage unit 61, a first feature mapping unit 62, a feature selection unit 63, a second feature mapping unit 64, and label correlation classification The feature selection unit 65 specifically includes an objective function obtaining module 631, an objective function solving module 632, a projected example removal module 633 and a reference space obtaining module 634, and the label correlation classifier unit 65 specifically includes a linear decision function module 651 , a correlation matrix definition module 652 , a classifier objective function module 653 and a label correlation classifier obtaining module 654 .
[0120] Among them, the known ...
PUM
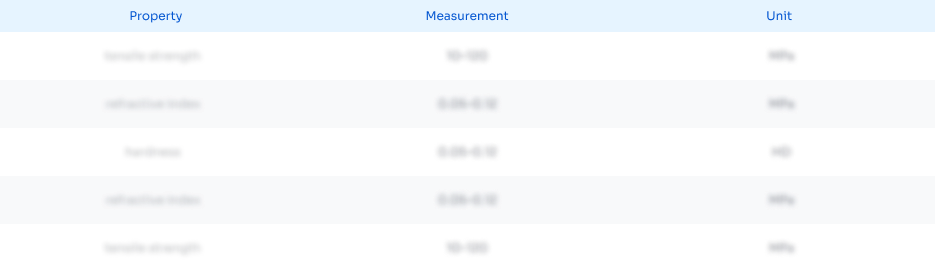
Abstract
Description
Claims
Application Information
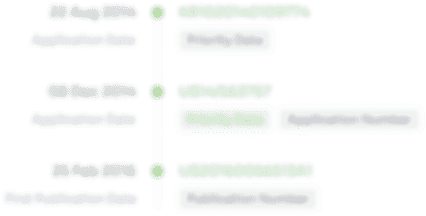
- R&D Engineer
- R&D Manager
- IP Professional
- Industry Leading Data Capabilities
- Powerful AI technology
- Patent DNA Extraction
Browse by: Latest US Patents, China's latest patents, Technical Efficacy Thesaurus, Application Domain, Technology Topic, Popular Technical Reports.
© 2024 PatSnap. All rights reserved.Legal|Privacy policy|Modern Slavery Act Transparency Statement|Sitemap|About US| Contact US: help@patsnap.com