Cross-media search method based on isomorphic subspace mapping and optimization
A homogeneous subspace and cross-media technology, applied in the field of cross-media retrieval based on homogeneous subspace mapping and optimization, to achieve the effect of good retrieval efficiency
- Summary
- Abstract
- Description
- Claims
- Application Information
AI Technical Summary
Problems solved by technology
Method used
Image
Examples
Embodiment 1
[0067] Such as figure 1 As shown, the cross-media retrieval method based on isomorphic subspace mapping and optimization in this embodiment, its specific steps are as follows:
[0068] The first step, isomorphic subspace mapping based on audiovisual feature analysis
[0069] The underlying content features of different types of multimedia data are extracted, and the correlation-preserving mapping is performed in the high-dimensional kernel space to obtain the isomorphic subspace Z.
[0070] (1) Extract three visual features of color histogram, color aggregation vector and Tamura directionality from the image database to obtain the visual feature matrix A;
[0071] Extract the four auditory features of centroid, attenuation cut-off frequency, spectral flow and root mean square from the audio database, and use the method of fuzzy clustering to index the auditory features, and unify the auditory features of each audio sample to the same dimension, Get the auditory feature matri...
Embodiment 2
[0114] A method for cross-media retrieval based on isomorphic subspace mapping and optimization. as attached figure 2 As shown, taking the "explosion" audio clip as a query example to perform cross-media retrieval, the specific steps are as follows:
[0115] The first step, isomorphic subspace mapping based on audiovisual feature analysis
[0116] The underlying content features of different types of multimedia data are extracted, and the correlation-preserving mapping is performed in the high-dimensional kernel space to obtain the isomorphic subspace Z.
[0117] (1) Collect image database and audio database, including the following 8 different semantic categories: explosion, airplane, lightning, insect, car, dog, monkey, elephant, each category includes 80 images and 40 audio segments; Extract the three visual features of color histogram, color aggregation vector and Tamura directionality from the database, and obtain the visual feature matrix A, where the image samples of...
PUM
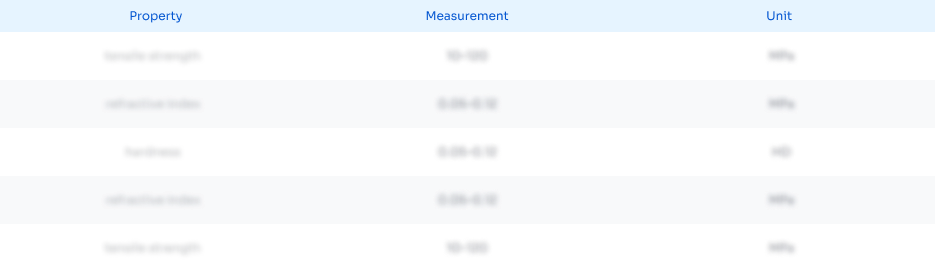
Abstract
Description
Claims
Application Information
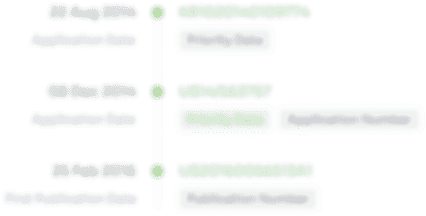
- R&D Engineer
- R&D Manager
- IP Professional
- Industry Leading Data Capabilities
- Powerful AI technology
- Patent DNA Extraction
Browse by: Latest US Patents, China's latest patents, Technical Efficacy Thesaurus, Application Domain, Technology Topic, Popular Technical Reports.
© 2024 PatSnap. All rights reserved.Legal|Privacy policy|Modern Slavery Act Transparency Statement|Sitemap|About US| Contact US: help@patsnap.com