Sparse representation features for speech recognition
一种语音识别、语音的技术,应用在语音识别、语音分析、仪器等方向,能够解决无法先验地获知类别或类型等问题,达到提高语音识别性能的效果
- Summary
- Abstract
- Description
- Claims
- Application Information
AI Technical Summary
Problems solved by technology
Method used
Image
Examples
Embodiment Construction
[0012] The principles of the invention will be described herein in the context of exemplary embodiments of methods, apparatus, articles of manufacture and systems for providing speech recognition functionality. It should be understood, however, that the principles of the present invention are not limited to the particular methods, apparatus, articles of manufacture and systems exemplarily shown and described herein. Rather, the principles of the present invention relate broadly to speech recognition techniques by which recognition performance can be improved by generating and using sparsely represented features in an example-based training approach. To this end, numerous modifications may be made to the illustrated embodiment within the scope of the invention. That is, no limitation to the specific embodiments described herein is intended or should be inferred.
[0013] It has been recognized that the failure of existing example-based approaches to the recognition task can be...
PUM
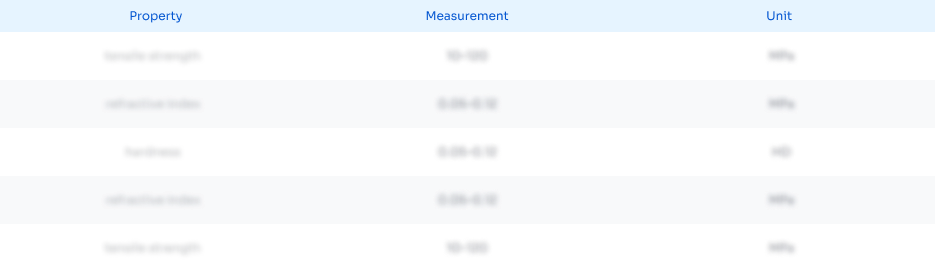
Abstract
Description
Claims
Application Information
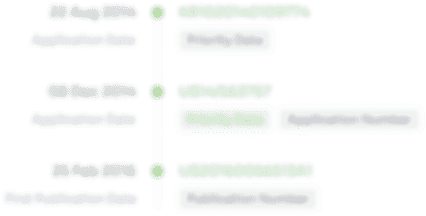
- R&D Engineer
- R&D Manager
- IP Professional
- Industry Leading Data Capabilities
- Powerful AI technology
- Patent DNA Extraction
Browse by: Latest US Patents, China's latest patents, Technical Efficacy Thesaurus, Application Domain, Technology Topic, Popular Technical Reports.
© 2024 PatSnap. All rights reserved.Legal|Privacy policy|Modern Slavery Act Transparency Statement|Sitemap|About US| Contact US: help@patsnap.com