Backward learning-based dynamic multi-attribute service selecting method
A service selection, multi-attribute technology, applied in special data processing applications, instruments, electrical digital data processing, etc., can solve the problem of increasing user difficulty, difficult to adapt to system function self-adaptation, pre-judgment, without considering service operation history information And other issues
- Summary
- Abstract
- Description
- Claims
- Application Information
AI Technical Summary
Problems solved by technology
Method used
Image
Examples
Embodiment Construction
[0040] This example is to use this method in a home surrounding intelligent environment. An implementation system of this method is designed based on the Java platform and Jena2.6.2 in the home-surrounding intelligent environment. The ontology and reasoning rules adopt HOAO and HOAO-R. The system has the semantic matching reasoning ability of service functional attributes and non-functional attributes.
[0041] Ⅰ. User preference learning based on backward learning
[0042] In the surrounding intelligent environment of the home, multiple users use various services provided in the surrounding intelligent environment through personal mobile terminals, and learn the user's preference habit by invoking and evaluating a centralized service of a candidate service. The flowchart of user preference learning based on backward learning is as follows: figure 1 shown.
[0043] Ⅰ-i. Register multiple candidate services in the service registry as a learning set.
[0044] Construct user p...
PUM
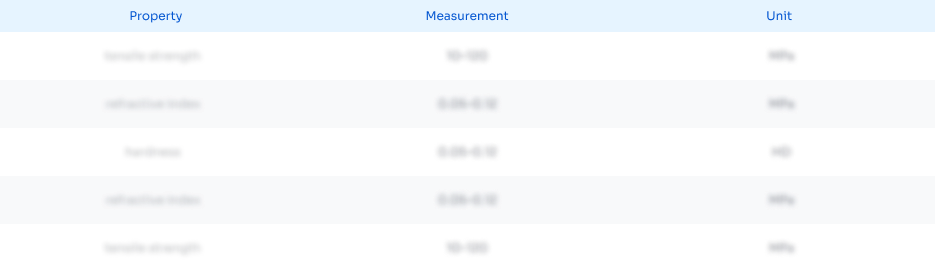
Abstract
Description
Claims
Application Information
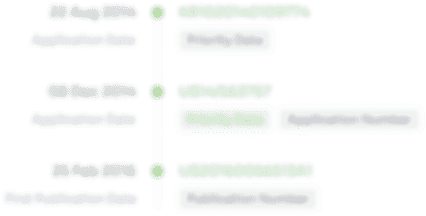
- R&D Engineer
- R&D Manager
- IP Professional
- Industry Leading Data Capabilities
- Powerful AI technology
- Patent DNA Extraction
Browse by: Latest US Patents, China's latest patents, Technical Efficacy Thesaurus, Application Domain, Technology Topic, Popular Technical Reports.
© 2024 PatSnap. All rights reserved.Legal|Privacy policy|Modern Slavery Act Transparency Statement|Sitemap|About US| Contact US: help@patsnap.com