Nonlinear laser fluorescence spectrum real-time identification method
A fluorescence spectrum and recognition method technology, applied in the field of real-time recognition of nonlinear laser fluorescence spectrum, can solve problems such as no public reports, and achieve the effects of less training samples, shortened computing time, and reduced the number of support vectors
- Summary
- Abstract
- Description
- Claims
- Application Information
AI Technical Summary
Problems solved by technology
Method used
Image
Examples
Embodiment 1
[0045] figure 1 It is a flow chart of the present invention. First, 30 groups of fluorescence spectrum data with five types of oil labels are selected from the oil product library as the learning sample spectrum, and 3 groups of laser fluorescence spectrum data of unknown oil products are actually measured as the test sample spectrum.
[0046] image 3 , Figure 4It is the initial fluorescence spectrum diagram with light diesel oil logo and lubricating oil logo in the spectra of 30 learning samples respectively, extracting effective fluorescence bands as the region of interest ROI, the process of extracting the region of interest is to scan line by line from the data head, If saturation and empty data are encountered, delete this part of the band, and finally obtain spectral data containing only fluorescence information. And normalize its intensity in the range of [0, 1], the entire extracted spectrum is characterized by five bands from 0 to 4 bands, as a preprocessing lear...
Embodiment 2
[0057] Embodiment 2 uses image data to classify, and preprocesses the sample image after ROI selection of the region of interest: the image is converted into a binary image, and the background is set to 0, and the spectral feature is set to 1, and then the image size is uniformly transformed into p x p. figure 2 Shown is the process of spectral image position offset correction, scan the preprocessed image sequence frame by frame, traverse all sub-blocks, check whether the image position exceeds the offset value, if the image position offset is too large, redo the region of interest ROI Select and continue to check until the offset condition is met, continue to perform curvelet transformation, input support vector base training, and finally get the classification result.
PUM
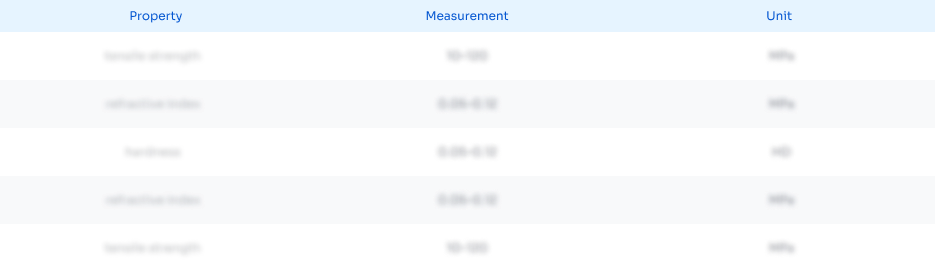
Abstract
Description
Claims
Application Information
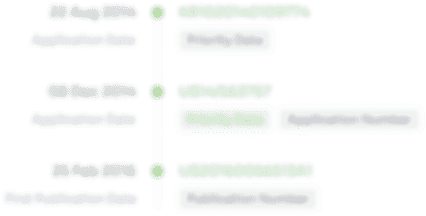
- R&D Engineer
- R&D Manager
- IP Professional
- Industry Leading Data Capabilities
- Powerful AI technology
- Patent DNA Extraction
Browse by: Latest US Patents, China's latest patents, Technical Efficacy Thesaurus, Application Domain, Technology Topic, Popular Technical Reports.
© 2024 PatSnap. All rights reserved.Legal|Privacy policy|Modern Slavery Act Transparency Statement|Sitemap|About US| Contact US: help@patsnap.com