Protein secondary structure engineering prediction method based on large margin nearest central point
A secondary structure and prediction method technology, applied in special data processing applications, instruments, electrical digital data processing, etc., can solve problems such as low prediction efficiency, local minimum value of data weights, etc., and achieve fast and efficient prediction effects
- Summary
- Abstract
- Description
- Claims
- Application Information
AI Technical Summary
Problems solved by technology
Method used
Image
Examples
specific Embodiment approach 1
[0018] Specific implementation mode one: the following combination figure 1 , figure 2 This embodiment will be specifically described. An engineering prediction method of protein secondary structure based on the nearest central point of large interval, which is realized by the following steps:
[0019] Step 1. Download the published NCBI nr database and protein structure data in PDB format, and construct a non-redundant protein secondary structure training data set based on the protein structure data in PDB format;
[0020] Step 2, given the primary sequence data of the target protein, constructing a multiple sequence alignment feature vector for each residue in the primary sequence of the target protein according to the NCBI nr database provided in step 1;
[0021] Step 3. Based on the multiple sequence alignment eigenvector of the target protein sequence constructed in step 2, call the large interval nearest center point algorithm to obtain the secondary structure predict...
specific Embodiment approach 2
[0074] Specific embodiment 2: This embodiment is a further description of the engineering prediction method of protein secondary structure based on the nearest central point of the large interval described in the specific embodiment 1. The initial hyperparameters described in step 3.3 The value range of μ is 0, 0.1, 1, 5, 10 or 20, and the optimal value of the hyperparameter μ within the range is quickly determined by using the RS126 non-redundant data set.
[0075] Since the PDB data training set derived from the PDB database described in step 1 contains quite a lot of protein chains, the subgradient projection algorithm of the PDB data training set takes a long time to converge. Therefore, the RS126 non-redundant data set is used to quickly determine the hyperparameter μ, and the hyperparameter μ described in this embodiment is used to regularize the linear transformation matrix. Selecting an appropriate hyperparameter μ can prevent over-learning and prevent the learned mode...
specific Embodiment approach 3
[0076] Specific implementation mode three: the following combination image 3 This embodiment will be specifically described. This embodiment is a further limitation of the method for engineering prediction of protein secondary structure based on the nearest central point of a large interval described in the first embodiment. In step 1, constructing a non-redundant protein secondary structure training data set is Achieved by following steps:
[0077] Step 1.1. Based on the protein structure data in PDB format determined by X-ray crystal diffraction released in the PDB database, apply the DSSP program to convert the protein structure data in PDB format into a data file in DSSP format;
[0078] Step 1.2: Convert the data file in DSSP format into a protein sequence data file in FASTA format based on the definition of DSSP format. At the same time, the 8 secondary structures defined by DSSP are classified into 3 types, among which, the H conformation, G conformation, and I confo...
PUM
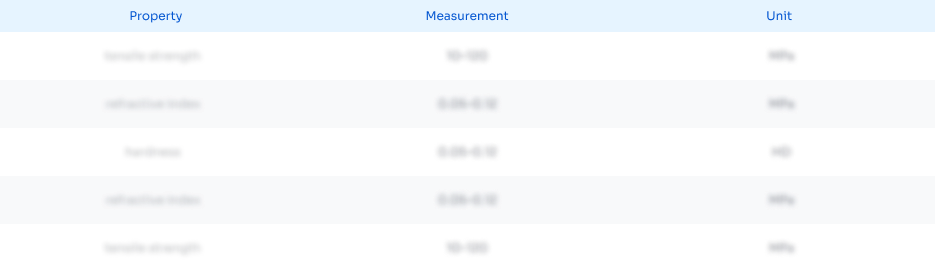
Abstract
Description
Claims
Application Information
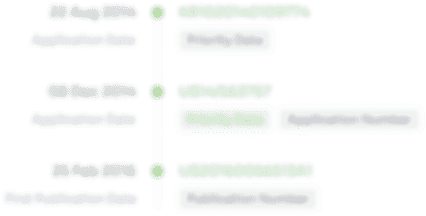
- R&D Engineer
- R&D Manager
- IP Professional
- Industry Leading Data Capabilities
- Powerful AI technology
- Patent DNA Extraction
Browse by: Latest US Patents, China's latest patents, Technical Efficacy Thesaurus, Application Domain, Technology Topic, Popular Technical Reports.
© 2024 PatSnap. All rights reserved.Legal|Privacy policy|Modern Slavery Act Transparency Statement|Sitemap|About US| Contact US: help@patsnap.com