Knowledge pushing method and system for scientific research tasks
A technology of knowledge push and task, applied in the field of knowledge push and system for scientific research tasks, can solve problems such as low accuracy, inability to achieve accurate push of scientific research task knowledge, single algorithm, etc., to achieve the effect of improving work efficiency
- Summary
- Abstract
- Description
- Claims
- Application Information
AI Technical Summary
Problems solved by technology
Method used
Image
Examples
Embodiment 1
[0037] A knowledge push method for scientific research tasks, first classify tasks, and then describe user portraits according to different task classifications, set corresponding portrait labels for each user, and tag specific tasks under a certain category. ; For specific tasks, a hybrid similarity algorithm is used to implement knowledge push to users; the hybrid similarity algorithm includes a tag matching algorithm and a text similarity algorithm, and the recommended results of the tag matching algorithm and the text similarity algorithm are combined, and the tags The products of the recommendation scores of the matching algorithm and the text similarity algorithm and the preset weight are added together to obtain the recommended list and the recommendation score of the hybrid similarity algorithm respectively correspondingly.
[0038] Further, the weights of the label matching algorithm and the text similarity algorithm are respectively 0.6 and 0.4, then the recommendatio...
Embodiment 2
[0041] This embodiment is optimized on the basis of Embodiment 1, and the formula of the recommendation score calculated by the tag matching algorithm is as follows:
[0042]
[0043] where: N(u, i) represents the task u and knowledge i shared labels,
[0044] ω uk represent tasks u with tags k The relevance degree of , that is, the weight of the label relative to the task;
[0045] r ki Indicates the label k with knowledge i The relevance degree of , that is, the weight of the label relative to the knowledge.
[0046] Further, in the tag matching algorithm, the number of matching tags and the tag weight are considered to improve the accuracy of the recommendation result, and the tag weight is determined according to the order of the tags.
[0047] The other parts of this embodiment are the same as those of Embodiment 1, and thus are not repeated here.
Embodiment 3
[0049] This embodiment is optimized on the basis of Embodiment 1 or 2. In the text similarity algorithm, the task is firstly represented by text, the corresponding task text feature vector and knowledge document feature vector are generated, and then the cosine similarity The algorithm obtains the knowledge recommendation list and the recommendation score corresponding to each knowledge.
[0050] Further, the value of each attribute field of the task, as well as the text content of the task name and task description are grouped together, and then the keywords are extracted by the TF-IDF algorithm and the occurrence frequency of the keywords is calculated, which is represented by a vector; for any given The two space vectors A and B of , the cosine similarity θ is calculated by the dot product and the vector length, the formula is as follows:
[0051]
[0052] of which: A i , B i represent the components of each dimension of vector A and B, respectively;
[0053] Then, th...
PUM
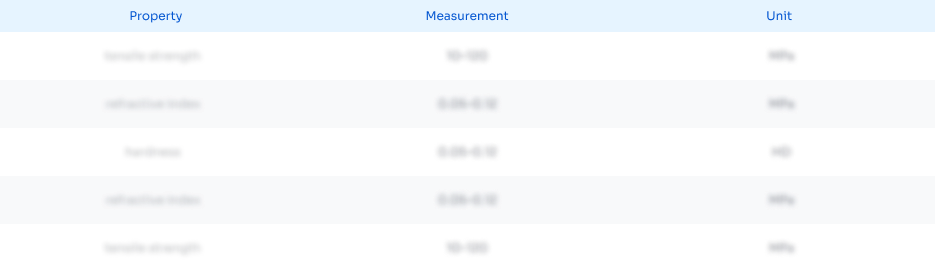
Abstract
Description
Claims
Application Information
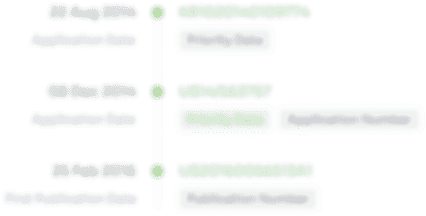
- Generate Ideas
- Intellectual Property
- Life Sciences
- Materials
- Tech Scout
- Unparalleled Data Quality
- Higher Quality Content
- 60% Fewer Hallucinations
Browse by: Latest US Patents, China's latest patents, Technical Efficacy Thesaurus, Application Domain, Technology Topic, Popular Technical Reports.
© 2025 PatSnap. All rights reserved.Legal|Privacy policy|Modern Slavery Act Transparency Statement|Sitemap|About US| Contact US: help@patsnap.com