A face recognition method and system
A face recognition and labeling technology, applied in the field of face recognition, can solve the problems of weak identification ability and CRC identification performance decline, and achieve the effect of increasing robustness, expanding quantity, and increasing identification ability.
- Summary
- Abstract
- Description
- Claims
- Application Information
AI Technical Summary
Problems solved by technology
Method used
Image
Examples
Embodiment 1
[0046] The embodiment of the present invention provides a face recognition method, such as Figure 1-2 shown, including the following steps:
[0047] (1) Extract the feature vector of the labeled face image training set to obtain the first feature vector set, and X n The class label of is known, assuming that the class label of n labeled face images is L={l 1 ,…,l i ,…,l n }, where l i is the label of the ith sample, and l i ∈{1,2,…,c}, c represents the total number of categories;
[0048] (2) Extract the feature vector of the unlabeled face image training set to obtain the second feature vector set,
[0049] (3) The label propagation algorithm based on collaborative representation is performed on the first feature vector set and the second feature vector set to obtain the soft label information of the unlabeled face image training set;
[0050] (4) Based on the soft label information of the unlabeled face image training set and the hard label information of the lab...
Embodiment 2
[0075] Based on the same inventive concept as Embodiment 1, an embodiment of the present invention provides a face recognition system, including:
[0076] The first feature extraction module is used to extract the feature vector of the labeled face image training set to obtain the first feature vector set;
[0077] The second feature extraction module is used to extract the feature vector of the unlabeled face image training set to obtain the second feature vector set;
[0078] a first computing module, configured to perform a collaborative representation-based label propagation algorithm on the first feature vector set and the second feature vector set, to obtain soft label information of the unlabeled face image training set;
[0079] The second computing module is used to calculate the inter-class scatter and intra-class scatter of all face image training sets according to the soft label information of the unlabeled face image training set and the hard label information of ...
PUM
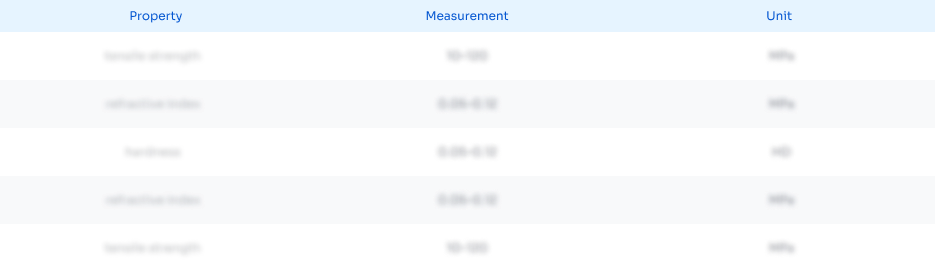
Abstract
Description
Claims
Application Information
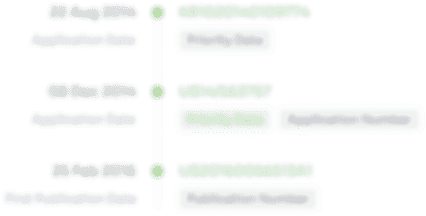
- R&D Engineer
- R&D Manager
- IP Professional
- Industry Leading Data Capabilities
- Powerful AI technology
- Patent DNA Extraction
Browse by: Latest US Patents, China's latest patents, Technical Efficacy Thesaurus, Application Domain, Technology Topic.
© 2024 PatSnap. All rights reserved.Legal|Privacy policy|Modern Slavery Act Transparency Statement|Sitemap