Self-adaptive fuzzy Kalman estimation SOC algorithm
An adaptive fuzzy, extended Kalman technology, applied in computing, computer-aided design, complex mathematical operations, etc., can solve the problems of obtaining SOC, difficult to reflect the real state of SOC, SOC jump, etc., to avoid SOC jump Effect
- Summary
- Abstract
- Description
- Claims
- Application Information
AI Technical Summary
Problems solved by technology
Method used
Image
Examples
Embodiment Construction
[0015] An adaptive Kalman Fuzzy SOC estimation algorithm, comprising the steps of:
[0016] Sl, third-order equivalent circuit model of a battery, such as figure 1 , The application extended Kalman algorithm to estimate the state variables, comprising a short voltage polarization, when the polarization voltage, the polarization voltage of the battery state of charge when the SOC long, state space equations and observation equations are as follows:
[0017] x (k) = A · x (k-1) + B · I bat (K) + v (k) (1)
[0018] V term (K) = C · x (k) + R 0 · I bat (K) + w (k) (2)
[0019]
[0020]
[0021] τ st = R st · C st
[0022] τ mt = R mt · C mt
[0023] τ lt = R lt · C lt
[0024] Wherein the current time k, k-1 is the previous time, x is the state variable, V oc Search for the open circuit voltage OCV-SOC SOC, S is the battery SOC, V term Real time measurement of the terminal voltage, R 0 The internal resistance of the battery, I bat (K) at time k for the charge and discharge curr...
PUM
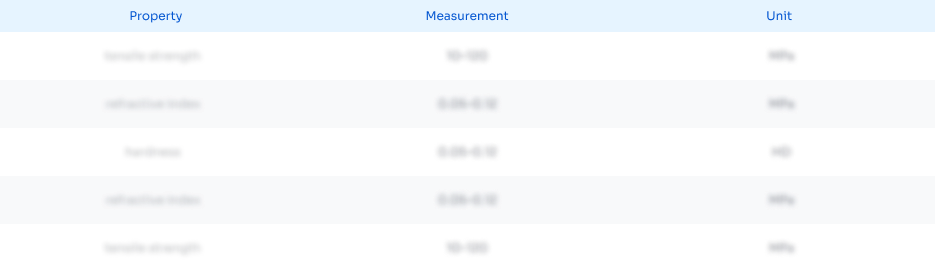
Abstract
Description
Claims
Application Information
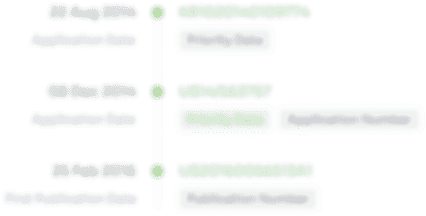
- R&D Engineer
- R&D Manager
- IP Professional
- Industry Leading Data Capabilities
- Powerful AI technology
- Patent DNA Extraction
Browse by: Latest US Patents, China's latest patents, Technical Efficacy Thesaurus, Application Domain, Technology Topic, Popular Technical Reports.
© 2024 PatSnap. All rights reserved.Legal|Privacy policy|Modern Slavery Act Transparency Statement|Sitemap|About US| Contact US: help@patsnap.com