Depth learning-based road network traffic situation forecast method and system
A traffic situation, deep learning technology, applied in the direction of road vehicle traffic control system, traffic control system, traffic flow detection, etc., can solve problems such as poor prediction accuracy and portability
- Summary
- Abstract
- Description
- Claims
- Application Information
AI Technical Summary
Problems solved by technology
Method used
Image
Examples
Embodiment Construction
[0139] The present invention will be further described below in conjunction with the accompanying drawings.
[0140] refer to Figure 1 to Figure 5 , a road network traffic situation prediction method based on deep learning, including the following steps:
[0141] S1, obtain multi-source traffic data and road network static configuration information, construct traffic flow parameter model; Described obtain multi-source traffic data, comprise Internet link speed data (Gaode map API, Baidu map API), detector flow data ( SCATS detector), traffic signal control program data; the static configuration information of the road network, including road network spatial geographic location information, intersection number, road section grade, road section length, road section number, lane number, lane function, etc.;
[0142] S2. Analyze the correlation of road network congestion and build basic forecasting groups;
[0143] S3. Construct a deep learning traffic situation prediction mode...
PUM
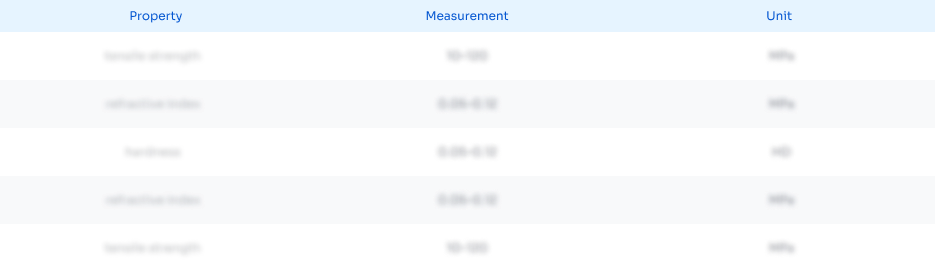
Abstract
Description
Claims
Application Information
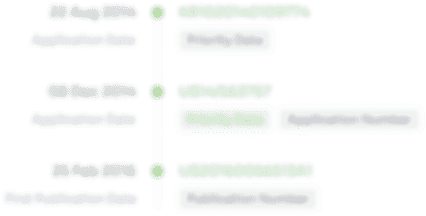
- R&D Engineer
- R&D Manager
- IP Professional
- Industry Leading Data Capabilities
- Powerful AI technology
- Patent DNA Extraction
Browse by: Latest US Patents, China's latest patents, Technical Efficacy Thesaurus, Application Domain, Technology Topic, Popular Technical Reports.
© 2024 PatSnap. All rights reserved.Legal|Privacy policy|Modern Slavery Act Transparency Statement|Sitemap|About US| Contact US: help@patsnap.com