Training system for automatic drive controlling strategies
A technology for automatic driving control and training systems, applied in the field of training systems, can solve problems such as high cost and danger
- Summary
- Abstract
- Description
- Claims
- Application Information
AI Technical Summary
Problems solved by technology
Method used
Image
Examples
Embodiment 1
[0044] First, through devices such as traffic cameras, high-altitude cameras, or drones, take road videos of road vehicles, pedestrians, and non-motor vehicles in a variety of different road scenes;
[0045] Secondly, the dynamic factors in the road video are detected by manual labeling method or object detection algorithm, and the position sequence of each dynamic factor is constructed;
[0046] Then, the sequence of positions of the dynamic elements is played in the simulator, ie the activity trajectory of the dynamic elements is generated.
Embodiment 2
[0048] Embodiment 1 is to replay the action trajectory of the dynamic factors shot in the simulator. There are two defects in this approach. First, the road scene of the simulator must be consistent with the scene shot in the video. Second, the dynamic factors do not have the environment Responsiveness is simply history replaying. An improved scheme based on machine learning methods is described below.
[0049] First, take road videos through traffic cameras, high-altitude cameras, drones and other devices;
[0050]Secondly, the dynamic factors in the road video are detected by manual labeling or object detection algorithms;
[0051] Then, for each dynamic factor o, its surrounding information S(o,t) at each moment t is extracted (surrounding information includes 360-degree visible static factors around the factor and other dynamic factor information, etc.), location information L(o ,t), and pair the surrounding information S(o,t) with the location movement information L(o,t...
approach example 2
[0068] [Scheme Example 2] Simulator transfer correction migration.
[0069] First, execute the control action sequence (a1, a2, a3, ..., an) on the unmanned vehicle entity, and collect the perception state (s0, s1, s2, s3, ..., sn) after each action is executed.
[0070] Secondly, in the simulator, the initial state is set to s0, and the same action sequence (a1, a2, a3,..., an) is executed to collect the perceived state (s0, u1, u2, u3,..., un).
[0071] Then, the constructor g is used to correct the deviation of the simulator. The function g inputs the current state s and the action a=π(s) given by the control strategy π, outputs the corrected action a' that replaces the action a, and actually executes the action a' in the environment, that is, a'=g(s,a ).
[0072] Thirdly, using evolutionary algorithm or reinforcement learning method to train g, the goal is to make the unmanned vehicle entity data and the data generated by the simulator as similar as possible, that is, to...
PUM
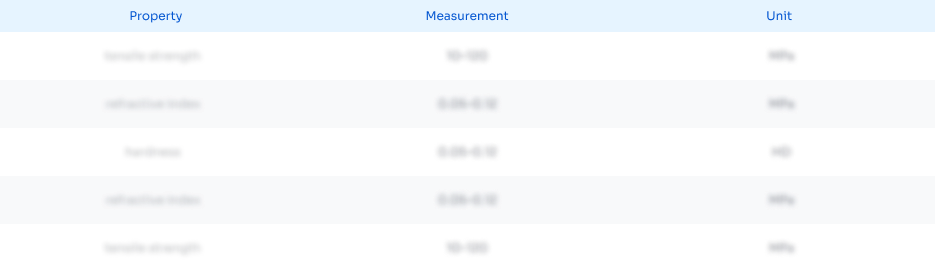
Abstract
Description
Claims
Application Information
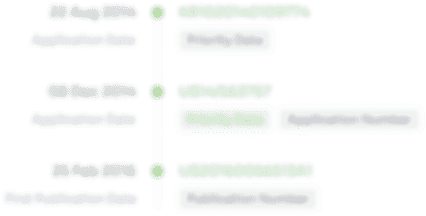
- R&D
- Intellectual Property
- Life Sciences
- Materials
- Tech Scout
- Unparalleled Data Quality
- Higher Quality Content
- 60% Fewer Hallucinations
Browse by: Latest US Patents, China's latest patents, Technical Efficacy Thesaurus, Application Domain, Technology Topic, Popular Technical Reports.
© 2025 PatSnap. All rights reserved.Legal|Privacy policy|Modern Slavery Act Transparency Statement|Sitemap|About US| Contact US: help@patsnap.com