Object-based OBIA-SVM-CNN remote sensing image classification method
A technology of OBIA-SVM-CNN and RBF-SVM, which is applied in the direction of instruments, character and pattern recognition, and computer parts, can solve the problems of low accuracy of remote sensing classification and recognition, and improve the accuracy of remote sensing classification, improve classification accuracy, The effect of overall classification accuracy improvement
- Summary
- Abstract
- Description
- Claims
- Application Information
AI Technical Summary
Problems solved by technology
Method used
Image
Examples
specific Embodiment approach 1
[0022] Specific implementation mode one: the specific process of the object-based OBIA-SVM-CNN remote sensing image classification method in this implementation mode is as follows: figure 1 shown.
[0023] Step 1. Segment the remote sensing image based on the multi-scale segmentation algorithm until all the segmented objects match the border of the farmland patch on the remote sensing image by visual inspection;
[0024] Step 2, train the RBF-SVM model and the CNN model, and obtain the trained RBF-SVM model and the CNN model;
[0025] Step 3. Use the trained RBF-SVM model and CNN model to classify and predict each segmented object in step 1 (for example, whether the segmented object is a farmland patch or a non-farmland patch), and obtain the CNN model prediction result and the SVM model forecast result;
[0026] Step 4: Carry out decision-making fusion of the prediction results of the CNN model and the prediction results of the SVM model to obtain the final classification r...
specific Embodiment approach 2
[0027] Specific embodiment 2: The difference between this embodiment and specific embodiment 1 is that in the first step, the remote sensing image is segmented based on the multi-scale segmentation algorithm, until all the segmented objects and farmland patches on the remote sensing image are visually inspected Boundary matching; the specific process is:
[0028] The segmented object is a farmland patch;
[0029] With the support of Yikang software, a multi-resolution segmentation algorithm (multi-resolution segmentation, MRS) is used to segment remote sensing images into farmland patches (objects) with homogeneous spectral and spatial information;
[0030] The multi-scale segmentation algorithm contains three segmentation control parameters, namely scale (scale), color measure (color) and smoothness measure (smoothness);
[0031] Determine the optimal combination of segmentation control parameters by trial and error (manually adjust the scale, color scale, and smoothness sca...
specific Embodiment approach 3
[0033] Specific embodiment three: the difference between this embodiment and specific embodiment one or two is that in the step two, the RBF-SVM model and the CNN model are trained to obtain the trained RBF-SVM model and the CNN model; the specific process is :
[0034] Select the radial basis function (radial basis function, RBF) as the kernel function (RBF-SVM) of the support vector machine to establish the support vector machine model;
[0035] Select M training sample points with classification labels, and extract image features according to the training sample points (for example, the remote sensing images with classification labels are remote sensing images of farmland patches and non-farmland patches in known remote sensing images, or the classification labels are Dadu and corn) as the input of RBF-SVM model;
[0036] The penalty coefficient C and the kernel coefficient γ are used as parameters of the RBF-SVM model;
[0037] The training sample point is a pixel in the...
PUM
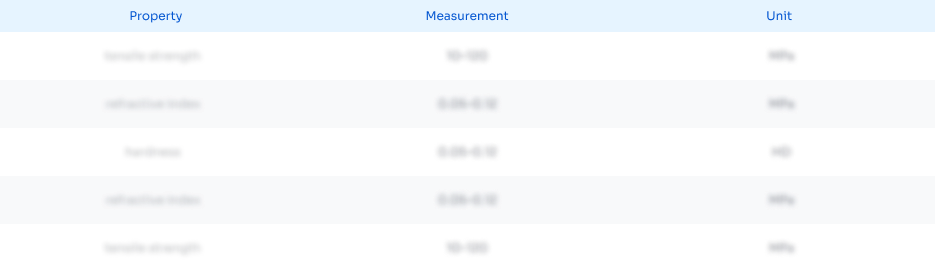
Abstract
Description
Claims
Application Information
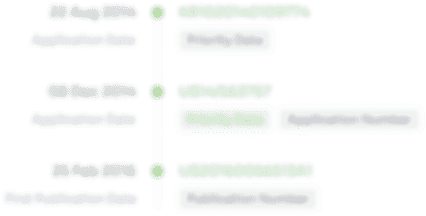
- R&D Engineer
- R&D Manager
- IP Professional
- Industry Leading Data Capabilities
- Powerful AI technology
- Patent DNA Extraction
Browse by: Latest US Patents, China's latest patents, Technical Efficacy Thesaurus, Application Domain, Technology Topic, Popular Technical Reports.
© 2024 PatSnap. All rights reserved.Legal|Privacy policy|Modern Slavery Act Transparency Statement|Sitemap|About US| Contact US: help@patsnap.com